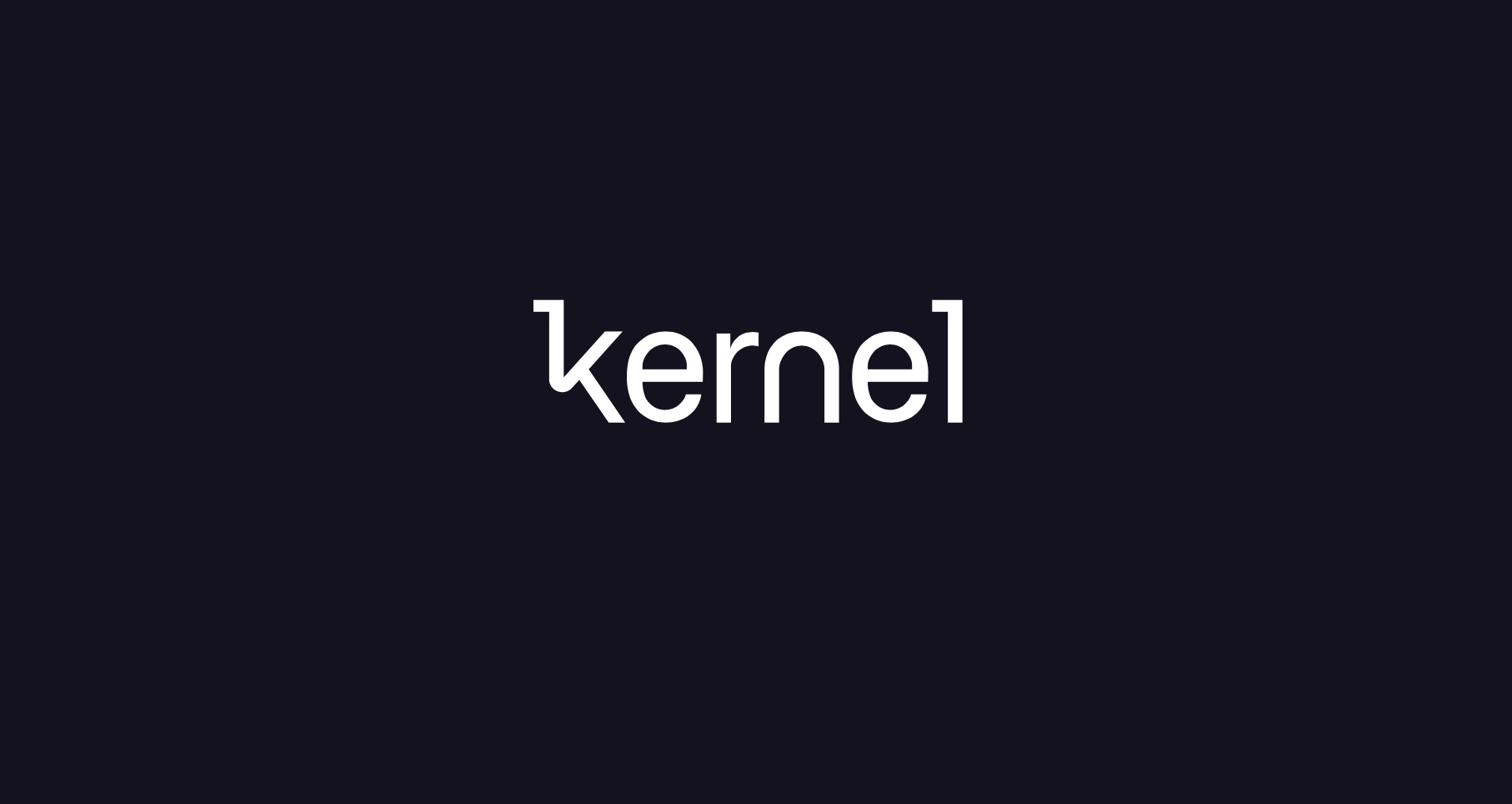
All views expressed in this article are my own.
This article is also available on Kernel's website.
I've got some news. In June of this year, I moved to sunny Los Angeles to join Kernel as a Data Scientist. I left academia (again) to join the small group of engineers, scientists, designers and many more who have banded together to change the world by democratizing neuroscience. In this post, I want to share why I joined Kernel and what I've learned in the six months since doing so. I hope to shed some light on what Kernel is attempting to achieve, and perhaps help others who are currently at a crossroads in their professional lives (especially early-career scientists and engineers). So let's get to it!
Why I joined
A compelling mission (hint: it's not about control)
Working in the field of neurotechnology for the last ten years has been an incredibly exciting and rewarding experience, offering a first-row seat to a major shift from academic research and science-fiction towards exciting companies and products, big and small. Perhaps even more notable is the way the field has captured the public's imagination, spurring a myriad of discussions and debates around a wide range of topics, from augmentation to brain uploading, all the way to the nature of consciousness itself.
This earnest effort to turn decades of neuroscientific research into compelling products has spawned a vast (and ever-increasing) number of startups. A sizable share of these privately funded efforts focus on control (e.g. Facebook, Neuralink, Paradromics). The idea is compelling—replace the computer mouse with a mere thought (or flick of the wrist)—but is also limiting. Control is a fairly narrow problem. And the bar is set very high (for non-medical applications). Superseding the intricate machinery that is the hand is a tall order. There certainly will be, and already are, some specific (often niche) use-cases where the benefits of a hands-free neural control signal can make up for most of the limitations, but I think truly mainstream adoption is still somewhere over the horizon. That's not to say I don't believe in a future where control will be entirely mediated by neural interfaces. I think it's inevitable. Rather, the current focus on control may be distracting us from more immediate, and perhaps more exciting, applications.
This is where Kernel comes in. Kernel is openly and intentionally not pursuing control. In fact, the very nature of Kernel's first device, Flow (a functional near-infrared spectroscopy helmet that uses light to measure hemodynamic changes in the brain), makes it a poor choice for replacing your mouse. Instead, Kernel is going after something else: quantifying the brain.
It's been famously said that "what gets measured gets improved." Without dwelling on the merits of that particular quote, it does outline an important fact: we are blind to what we cannot measure. If your doctor doesn't know your blood pressure, they can never hope to fix it. Similarly, counting your steps or measuring your resting heart rate can lead to significant improvements in fitness and health. Regrettably, when it comes to the brain, we measure very little. Sure, we have expensive and difficult to interpret medical imaging. But we lack simple, widespread and easy to understand measures of brain activity and health. The brain doesn't have the equivalent of a blood pressure cuff (i.e. something you might measure once or twice a year in the right setting, like your doctor's office ). And the brain most certainly doesn't have the equivalent of a fitness tracker (i.e. something you might use at home, every day).
That's fundamentally Kernel's ambition: measure something of substance about the brain non-invasively and distill it into a metric that's as simple as possible, yet still informative. Even a single brain measure fitting the above description would be a game-changer—enabling us to inform, entertain, improve, guide, learn and grow. That's a clear and exciting mission, and one I was immediately drawn to.
Openness and humility
As a scientist and lifelong skeptic, I have been fairly transparent about my dislike of the neurobabble and hyperbole that is prevalent in the neurotech industry. While I can certainly appreciate the necessity for embellishment, I think there's a fine line between sales tactics and plain old nonsense—one too many happily step over.
Since I first started following Kernel, I was struck by the openness and humility with which they approach their ambitious goals. I have watched as Kernel leaned into and cultivated this philosophy, and I now consider it to be one of its greatest strengths. Internally, this approach ensures that decisions are made rationally and that scientific rigor is embraced. Externally, it builds trust within the neurotech community and beyond and serves as a template for other companies to follow. This thinking is also reflected in Kernel's commitment to giving users full agency over what happens to their personal and brain data—a refreshing position in today's tech landscape.
A recent and compelling example of this philosophy at work is the Flow U program, designed to put Kernel's device in the hands of scientists for a free year-long trial. If researchers don't find value in what Kernel has to offer, they can return the device at no cost.
Importantly, while Kernel's approach is marked by openness and humility, it does not lack in determination when it sets its sights on a target. Nor does it shy away from taking credit for its successes—which have been remarkable, as we'll touch upon later. To me, this represents the perfect balance and allows Kernel to set groundbreaking, yet realistic, ambitions.
A word about the academia-industry dichotomy
I had resolved not to stoke the flames of this infamous debate any higher. But the thought that it might help others—including my younger self—set a course in their professional lives with more confidence made me reconsider.
Some say the differences between industry and academia are overplayed, yet my experience has been that of two consistently different worlds. A lot of the obvious distinctions have become well-worn clichés by now and need no repeating (i.e. the money, work-life balance, etc). There is one, however, which I have encountered less often, yet was the most meaningful for me personally: the level and depth of collaboration.
Companies offer a much more collaborative, open and sharing working environment. The best explanation for this I can think of is that academia is ultimately a single-player game, where you—the researcher—are the brand and the product. My experience outside of academia has been starkly different. The goal alignment intrinsic to laboring together towards a clearly articulated goal lends itself to much more spontaneous, frictionless and genuine collaboration—and on much larger scales. While this is certainly a matter of personal preference, the feeling of belonging and cooperation stemming from this type of working environment can be highly fulfilling, and frankly: a lot of fun.
Luck, opportunity, and the windiness of life's path
In addressing the question of "Why I joined Kernel," it would be disingenuous not to acknowledge the role played by luck and opportunity. Yet I think there is an important lesson in this too. Life is hard to predict and plan for. Things go awry, surprises happen (I'm looking at you COVID-19), and reality gently brushes your plans aside.
But amidst the noise of circumstance, it pays to be prepared and it certainly pays to be persistent. In hindsight, I can see that the path that led me to Kernel was a long spiral, rather than a straight line. When I first heard of Kernel's existence, several years ago, I immediately applied. While that disappointingly didn't work out, learning about Kernel's progress—and writing about it on this blog—ultimately put me on a collision course with them. Without necessarily knowing it, the choices I made along the way prepared me to seize the opportunity when it came. Like Steve Jobs famously said: "You can’t connect the dots looking forward; you can only connect them looking backwards. So you have to trust that the dots will somehow connect in your future."
What I learned
A really compelling mission
I've explained why Kernel's ambitious mission was so compelling to me from the outside looking in. What I've learned since joining the company has deepened my appreciation for it. The brain is an unimaginably complex object that we are only beginning to understand. Despite our relative ignorance, we can already measure a remarkable number of things about it using various physical sensing modalities (electrical, optical, magnetic, acoustic, etc). Amongst these non-invasive approaches, Kernel's Flow device stands out by using an advanced optical measuring technique (time-domain functional near-infrared spectroscopy, which I explored in more depth here) that gives it unique advantages over more commonly used methods (such as electrical-signal based EEG).
These precise measurements will allow Kernel to define simple yet informative metrics that quantify the brain's health and state. While a simple low-dimensional measure will never describe the full complexity of a system like the brain, there are clear advantages to breaking down large, difficult-to-probe systems into digestible insights. Some familiar examples of (relatively) complex systems being reduced to accessible metrics include: resting heart rate (an indicator of cardiovascular health), heart rate variability (an indicator of both cardiovascular health and autonomic nervous system function), and body-fat percentage (an indicator of fitness and adiposity). Each of these examples allows entire companies (or divisions) to exist and can be measured at home with affordable and simple to use devices (smart scales, fitness trackers, etc). What if we had a handful of metrics like that for the brain (our most important and perplexing organ)? For instance, what if we had a metric that captured brain performance/ability, and one that captured brain health/aging. Wouldn't you want to know these two numbers for your brain and how they compare to others in your age group, or finally be able to measure the impact of your life choices on its health and performance? I know I certainly would!
These rigorous, yet simple to understand metrics could form the basis of a wide range of compelling applications in areas as diverse as self-improvement (e.g. neurofeedback), entertainment (e.g. adaptive video-games), mental health (e.g. monitoring, virtual reality therapy, etc), learning (e.g. real-time state monitoring), and many more.
While the appeal for individuals is clear, the current price and form factor put that slightly out of reach (although as I've written in the past, Kernel's ambition is to rapidly democratize access to these insights by having a Flow device in every home by 2033). Luckily, the very same metrics I described above can provide tremendous value to individuals without ever putting a device on their heads. We live in the era of social media, targeted ads and behavioral nudging, all of which have profound—yet often poorly understood and measured—impacts on our brains. With the tools at Kernel's disposal, we have the opportunity to identify and communicate how a given activity or product impacts your brain, using simple, validated metrics rather than obscure, difficult to replicate ad-hoc studies. Think of it as identifying fast food for the brain so you can steer clear.
Taking this line of thought further, we can imagine how forward-thinking companies might agree to go through a certification process akin to an organic label for the brain, designed to verify that their experiences and products have a positive or neutral impact on the brain. Armed with this knowledge, consumers might begin making more deliberate choices about what they expose their brains to and companies might finally have the incentive to take mental health and wellbeing into account early in the design process.
All of this is easier said than done. But Kernel's strength isn't that it has all the answers (although we have some ideas 🤫). Rather, Kernel's strength is that it is currently the best-positioned player—by a long shot—to get to those answers. I'll expand on why I believe that to be the case next.
World-leading team and expertise
I've mentioned my affinity for the collaborative spirit of startup companies. At Kernel, this is magnified by the exceptionally talented individuals it is made up of. While my sample size is modest, I can safely say that I have never felt surrounded by as much competence and brilliance as I am at Kernel. One of the most difficult challenges a startup faces is assembling a world-class team while safeguarding its values and unique character. Kernel has done a tremendous job at this.
What is perhaps even more remarkable is that Kernel has achieved this as a full-stack company—one which deals in everything from circuit design, mechanical prototyping, and software engineering to machine learning, neuroscience, cloud analysis, and more. Very few places (companies or otherwise) can say they have a state-of-the-art device that is entirely designed, assembled, tested and then deployed as part of large scale, rigorous neuroscientific research studies—all in the same building.
The quality and breadth of expertise on Kernel's team, perhaps more than any other point I made in this post, is what gives me immense confidence in its ability to succeed.
A device so good that Kernel has no direct competition
Let's talk product. Up until now, I've focused on the intangible: the vision, the mission, the talent. But Kernel has been around for some time, and those qualities have already delivered some very tangible results—Flow.
By all conceivable metrics, Kernel Flow is leaps ahead of any other non-invasive optical system out there (i.e. number of channels, head coverage, sampling frequency, ease-of-use, scalability, etc). More importantly, Kernel has carved out a unique niche within the entire spectrum of non-invasive brain measuring techniques. In contrast to other common approaches, like EEG, Flow has greater spatial resolution and access to unique information, such as the absolute concentration of oxygenated hemoglobin in the cortex. The ability to tap into this rich information—for the entire head—is one of Kernel's key competitive advantages. I do not believe that EEG, especially low channel-count systems (e.g. frontal only, in-ear, etc) will be able to provide the paradigm shift that Kernel is angling for. The only non-invasive devices that can compete with the data Flow produces are multi-million-dollar fMRI machines, which have none of the ease-of-use, portability and scalability needed to truly democratize access to these insights.
On the other end of the spectrum, invasive techniques (which require some form of surgery to insert into the brain) can offer rich information and are uniquely poised to improve the lives of people with spinal cord injury and other forms of trauma and disease of the nervous system. However, when it comes to consumer applications, invasive interfaces' current inability to capture data for the entire cortex, and their obvious barriers to entry, put them on an altogether different—and longer-term—trajectory.
It's therefore clear that Kernel operates in a very unique space. Flow strikes a careful balance: it can capture rich information about the brain while remaining portable, affordable and scalable. Nobody else can quite say that today—Kernel has invented and engineered itself out of any direct competition.
As I've said in a previous post, being on the most promising path still doesn't guarantee success—our mission may turn out to be impossible altogether. What I hope to have imparted, however, is that Kernel is currently the one company in the world best positioned to harvest the fruits of decades of neuroscience and share them widely.
The long road ahead won't be easy
Lest I be labelled overly optimistic—perhaps even naive—let me finish with this. Kernel is a startup. And a startup is still a startup: an unlikely bet taken in pursuit of a better future one believes to have glimpsed. The wilder the future, the riskier the bet. And Kernel is after a pretty wild future.
Everyone at Kernel is well aware that the road that lies ahead is littered with unknowns. There will most certainly be stumbles and a few dead-ends, perhaps even difficult decisions to make—that's the cost of dreaming big.
Kernel's mission of democratizing brain measurement hinges on finding the intersection between rigorous neuroscience, clarity and mainstream appeal. That's a tall order. But I think nobody else has a better chance—and that's an exciting position to be in.
If the 20th century had the space race, the 21st will be marked by the unravelling of the mystery that is the human mind. But unlike the conquest of space, the race to harness the power of the brain is increasingly being run by private companies, not nations. It’s exciting to witness the tremendous progress happening in the field of neurotechnologies and the broad interest bubbling up around it. While last century’s space race is plastered across the front pages of most streaming apps (e.g. For All Mankind, The Right Stuff, First Man), an equally momentous shift is happening in neuroscience right now, albeit at the sluggish pace of real-time. In the era of binge-watching, the slow trickle of scientific advances and technological breakthroughs can leave one hungry for more, just like the abridged weekly episodes of a favourite show. Good thing, then, that a new episode has aired. The latest instalment in the neurotech saga comes to us courtesy of Kernel, Bryan Johnson’s neural interface company. Let’s press play.
Kernel debuted their first-ever device, Kernel Flow, during a recent live event. Kernel plans to make the Flow available to 50 lucky early partners in the coming months. If that device name sounds familiar, that’s because Kernel had unveiled it earlier this year (which I wrote about here). As a reminder, Kernel had announced two devices, the Flow, which we’ll talk more about in a minute, and the Flux, a magnetoencephalography (MEG) headset (note: I abhor acronyms, but I think we can all agree that a word like that deserves one). Bryan Johnson says the Flux MEG system is a mere 3 to 5 months behind its more mature sibling (Flow), so expect to hear more on that front soon.
A closer look at how Kernel Flow works
The star of the show was the Flow, a time-domain near-infrared spectroscopy (TD-NIRS) system (fine, maybe a few acronyms are okay). As the name suggests, NIRS uses infrared light to measure brain activity. The advantage of using light is that it’s safe (assuming certain limits are respected) and works from outside the body—no surgery required. Specifically, a source (a laser) pressed against the head shines light into the body. The light travels through the various layers (skin, bone, brain, etc), bouncing around like so many pinballs. Some portion of the light is absorbed by the body, while some of it makes it back out after lots of bouncing around and can be measured.
The principle behind NIRS can be demonstrated with a simple experiment most people are familiar with: shining light through the hand with a flashlight. When the light crosses the body, some of it is absorbed and some of it makes it to the other side. That’s why the light looks dimmer after passing through the hand. But why is it red? The answer is that light with a shorter wavelength (towards the purple side of the rainbow) is more strongly absorbed by the body, while the longer wavelengths pass through the body more easily (the red part of the rainbow). Although we cannot see it, infrared also passes through the skin very easily, which is one of the reasons it’s used for these types of measurement. Less absorption means infrared light travels deeper into the body and brings back more valuable information.
While shining light through the hand is an example of transmittance (light goes in on one side, comes out on the other), the human head is too large for that to work. Instead, NIRS relies on reflectance, which is achieved by placing light detectors near the source (e.g. arranged in a circle 1 cm around the laser). These sensors pick up light that bounced around inside the head (was scattered) and came back up to the surface. It’s possible to observe this effect with the same flashlight we shone through our hands earlier. By pressing it against any part of the body, the area around where the flashlight touches the skin will turn red. That’s reflectance.
If NIRS works by shining light into the body and measuring how much of it is reflected, how can it detect brain activity? To answer that, we must first look at an interesting optical property of blood. A major component of blood is hemoglobin, a large protein which binds to oxygen and carries it from the lungs to the rest of the body, where the oxygen is released and used as fuel by cells. Interestingly, hemoglobin absorbs red and infrared light differently depending on whether it’s carrying oxygen or not. And a lot of the body’s absorption of that part of the spectrum comes from hemoglobin. This makes it possible to determine how much oxygenated blood is currently running through a body part by simply looking at how much red and infrared light it absorbs.
This (finally) brings us to the brain. When a region of the brain is active (e.g. because it’s involved in executing some task) the neurons and local tissue consume more oxygen (and glucose). This triggers a relatively fast and specific increase in blood flow to that region, to resupply it with oxygen and other metabolites (this process is called the hemodynamic response). Within seconds, the blood flow to an active region of the brain increases, bringing with it more oxygenated hemoglobin (and more blood volume overall) and “washing out” the deoxygenated hemoglobin. The blood supply goes back to normal within about 15 seconds. When light travels through a region of the brain undergoing this change in blood supply, more infrared light is absorbed because of the higher amount of oxygenated blood in the tissue. This change in absorption is how NIRS indirectly measures brain activity: it simply detects the increase in blood supply caused by the activation of a chunk of brain.
Not to go too far down the rabbit hole here, but there’s one more important piece of information about how Kernel Flow works. So far I’ve described NIRS in general terms, but I began this section by saying that Kernel Flow is a TD-NIRS device. Surely I wouldn’t have added two letters to an acronym (which you know how I feel about by now) just for fun. The TD stands for time-domain: a specific variant of NIRS which relies on extremely short pulses of light (only a few picoseconds long). Because the pulses are much shorter than the time it takes for light to bounce around the body and resurface, we can consider all of the light to have left at the same time. We can then use that assumption to estimate how long any light picked up by the detector travelled for—its time of flight—since we know both when it left the source and when it arrived at the detector. Knowing the time of flight is incredibly valuable because it allows us to “sort” the light based on how long it spent inside the body. Light that came back very quickly—and therefore probably bounced only a few times through the skin or bone and never reached the brain—mostly contains irrelevant information (artifacts). On the other hand, the light that arrived later—and therefore travelled deeper into the brain—contains valuable information about neural activity. Since this “late” light inevitably had to travel through those superficial structures before reaching the brain, we can subtract the signal obtained from the “fast” light to remove most artifacts. This makes TD-NIRS very good at extracting neural information specifically from the brain and ignoring irrelevant parts of the signal from the skin and skull. The additional depth information also helps localize neural activity more precisely within the cortex.
A simulation performed by Kernel showing the likely path (black lines) taken by light arriving at the detector (blue dot) from the source (red dot). Light arriving at the detector quickly (left panels) doesn't travel deep into the tissue, while light arriving later is more likely to have penetrated deep into the tissue (image from Kernel).
There’s one last advantage to TD-NIRS: using two light sources and some smart tricks allows for absolute concentrations of oxygenated and deoxygenation hemoglobin to be measured, instead of just relative changes. We’ll touch on this last bit some more later.
Putting Kernel Flow into context
To understand what Kernel is up against, it’s important to know that despite being available for a long time, NIRS has failed to gain widespread adoption as a tool for neuroscientific research (studying the brains of infants and children being perhaps the only exception, mainly because of how safe and robust to movement NIRS is). It has been steadily growing in popularity but remains a relatively niche brain recording method. While some researcher might push back against this characterization, it’s safe to say that NIRS has not reached as wide of an audience as some of the other non-invasive brain-recording approaches. The main reason for this is that NIRS is often seen as slow, big, imprecise and expensive. Not the best list of adjectives for a brain-computer interface, especially not one that ought to eventually find its way into people’s homes. Kernel is out to change that perception.
When Kernel began looking at this problem about five years ago, they started with a clean slate. They looked at everything under the sun—every possible way to peer into the brain. “We looked at literally everything,” Bryan Johnson tells me. Having quickly concluded that invasive technologies were not going to allow for the rapid democratization of brain interfaces that Kernel wanted to pursue, the team eventually settled on NIRS (and MEG) as the two most promising modalities. They saw great potential in these two somewhat neglected methods for measuring brain activity. In both cases, Kernel foresaw they could tackle many of the challenges preventing wider adoption of these devices, which were mostly engineering, rather than scientific, in nature (i.e. current devices are large, expensive, etc).
Their work clearly paid off. Kernel Flow blows all current TD-NIRS systems out of the water. For instance, a common limitation for NIRS systems is how quickly they can react to incoming light, which is usually measured in photons per second. The best research systems described in the literature reach up to a few tens of millions of photons per second (see here and here for examples). Kernel’s design has already demonstrated 800 million photons per second, with plans to reach above a billion—two orders of magnitude more than the state-of-the-art. When compared to existing commercial devices, the difference is even larger. The Kernel Flow is also a very flexible solution: by relying on a smart modular design, the headgear can be configured with a single sensor or up to 52 for full head coverage. Kernel has yet to announce any pricing, but the Flow is expected to cost an order of magnitude less than comparable devices. While the initial cost is unlikely to put the device in the range of a Christmas gift quite yet, it will be a compelling entry point for researchers, considering what the device offers.
Between the significant technological advances and the benefits inherent to TD-NIRS, Kernel hopes to have pushed the state-of-the-art far enough to unlock a whole new wave of interesting use-cases. Which brings up the following important question.
What exactly are you supposed to do with a TD-NIRS device at home?
The Kernel Flow will undoubtedly offer researchers already working with NIRS a powerful new tool. It will most likely also convince some researchers currently using other brain-measuring devices to make the switch to NIRS. But selling devices to scientists isn’t Kernel’s long term plan. They want to bring neuroscience to the masses. This raises the crucial question: what does one do with a TD-NIRS device at home?
The answer, according to Bryan Johnson, is that they don’t know yet. While this might sound surprising at first, it is the most honest answer he could give. Nobody knows all the ways a product that doesn’t exist yet might be used, especially if that product is a platform for others to build on. The reason that Kernel is creating the tool in the first place is the belief, shared by many in the blossoming neurotech industry, that the use cases will come—once the devices are built. Judging by the backlash Neuralink (another neurotechnology company) received from the scientific community for promising the sun and the moon, one can see why the cautious approach might be more judicious for Kernel.
“Reducing friction,” is what Bryan Johnson tells me Kernel is about. And he has experience doing just that: his previous company, Braintree (which later bought the payment app Venmo), focused on fast, simple and frictionless monetary transactions, to great effect (Braintree was purchased by PayPal for $800 million). The hope is that by building a best-in-class device at an approachable price, the applications will build themselves. At first, Kernel plans to make the Flow available to a wide range of early-adopters interested in exploring the device’s capabilities (neuroscientists, entertainment, gaming and pharmaceutical companies, etc). Once clear and compelling use-cases emerge, Kernel will start distributing the device to consumers.
While Bryan Johnson deliberately withholds any strong opinions about specific “killer apps,” he has some ideas about the broad types of applications Kernel Flow might unlock in the near future. Quantification, he says, is the name of the game. By listening in on the neural symphony reverberating through our brains at any given moment, it might be possible to quantify abstract and often ill-defined concepts, like focus, cognitive load, aging, mental health, pain and a slew of others. This concept of quantification is bolstered by the observation that we humans are remarkably bad at perceiving the world as it really is, especially our own blind spots and biases. Putting numbers to otherwise subjective experiences might bring forth a new era of neuro-quantification and shared understanding, something Bryan Johnson explored in a 2018 post (an interesting read).
At least that’s the vision. In the more immediate future, quantifying brain activity might enable applications more limited in scope, like quantifying “cognitive performance” as it changes throughout the day or based on environmental factors. How did getting only a few hours of sleep last night impact my cognitive performance? What about that heavy meal or long walk? The same idea could be applied to several common activities and situations such as meditation, trying to focus on a task or learning. In the era of Fitbits, Apple Watches and smart scales, one can envision consumers having an appetite for something like that. But it’s hard to imagine how these examples alone could lead to the type of mass adoption Bryan Johnson pictures. After all, Fitbits have a nasty habit of finding their way into the forgotten gadgets drawer.
Another of the Kernel Flow’s strengths, stemming from the TD-NIRS technology it’s based on, is the ability to measure absolute concentrations of oxygenated and deoxygenated hemoglobin. This seemingly small detail enables robust comparisons between measures obtained in different people, different brain regions in a single person or even the same region over time. This is a key advantage over competing recording techniques and might enable interesting new use cases, including health-related ones (prevention, early disease markers, etc). However, as Kernel is well aware, stepping into the medical realm comes with additional delays and countless regulatory hurdles, which can slow down progress and blow up costs. That’s probably why Kernel says they have no immediate plans to seek regulatory approval for their device—but are likely to do so down the road, especially if there is a clear clinical application within reach.
You may have noticed that the list of applications does not include controlling things with your mind, something Neuralink is actively pursuing. Surely using your mind to play video games or control your computer constitute interesting use-cases? The reason those applications aren’t part of the discussion is that NIRS is fundamentally too slow for controlling most things with your mind, where the delay between intention and action needs to be less than about a tenth of a second. As we explored in detail above, NIRS measures an indirect effect of brain activity: changes in blood oxygenation and volume. The biological phenomena that cause these measurable blood changes take time—that’s a fairly inescapable biological reality. It’s like finding out if someone is hungry by waiting for them to eat—you’ll only be able to tell after the fact. Nonetheless, Bryan Johnson believes the naysayers are too quick to dismiss NIRS as fundamentally slow. And there certainly may be ways to speed things up by using smart tricks, especially with a fast device like the Flow. But despite these hypothetical speed gains, it’s doubtful that NIRS could be used for fast-paced input, like playing an online video game. Of course, NIRS would be a good candidate for less time-sensitive control tasks, like turning the lights on. However, as Bryan Johnson points out, direct motor control is unlikely to be the most exciting consumer application for non-invasive brain interfaces. Instead, measuring the state of the brain could make for more interesting scenarios—for instance, rather than simply switching the lights on and off, one might envision a system that automatically adapts the lighting in the room to optimize your focus or alertness.
Could Kernel really get a brain-computer interface in every US home by 2033?
The current Kernel Flow pricing starts at five thousand dollars for a basic configuration. Bryan Johnson estimates that the entry price could drop to as little as a few hundred dollars once it’s produced at high enough volumes. That would put the device well within reach of consumers. And if future iterations bring that price down even further, one can start to see where the 2033 figure comes from.
Given the constraints of a non-invasive device, I would argue that Kernel is doing everything right. They’ve chosen a realistic and unique path, and they’re pouring resources into building a best-in-class device. They’re approaching the broader question of consumer applications with candour, restraint and an open mind. But doing everything right does not guarantee success, and the biggest question mark remains use-cases. It seems to me like quantifying brain performance will not be quite enough to get a majority of people excited. It may be a viable business, sure, but not a device-in-every-home kind of deal.
There are countless cautionary tales of exquisitely engineered technologies failing to convince consumers. Magic Leap and Microsoft built groundbreaking augmented reality headsets, far surpassing what Google glass could do, yet nobody knows quite what to do with them. Or 3D printing, which was lauded as a solution to planned obsolescence and would have people printing their own forks at home by now, yet has so far only found a foothold in specific niches (certainly not as a widespread consumer device).
When I mention Magic Leap, Bryan Johnson answers that unlike augmented reality headsets, a brain-measuring device has inherent value that goes beyond interactive applications and experiences. Accessing the information hidden inside your skull, he argues, is intrinsically valuable—and therefore worth the effort. Even without compelling “entertainment” options, the device might still find other uses, such as providing medically relevant information or supporting positive life changes (better learning, higher focus, etc). While that may be true, I would caution that a functional brain imaging system, like the Flow, provides the most useful information when used within a specific and well-defined context. Just looking at a brain without knowing what it’s doing isn’t typically of much value. Users will presumably need to wear the device while engaged in specific tasks—and will therefore need a good reason to do so. This is not to say that there is no viable path for consumer adoption—but rather that the path has yet to be discovered. What we can say, based on the device’s performance compared to other non-invasive solutions, is that whatever that killer-app ends up being—it will likely run on Kernel’s Flow.
Bryan Johnson spoke of an inflection point, a moment where a particular technology reaches the threshold of “compelling-enough,” starting to make money, unlocking further technological advances and sales in a runaway virtuous cycle. He believes the first iteration of the Flow might push Kernel past that point and put them on an exponential curve of progress and profit. But it’s also possible that their first device, despite its merits, will fall just shy of that threshold. We’ll find out for sure in an upcoming episode. For now, as is regrettably too often the case, we are left with a cliffhanger.
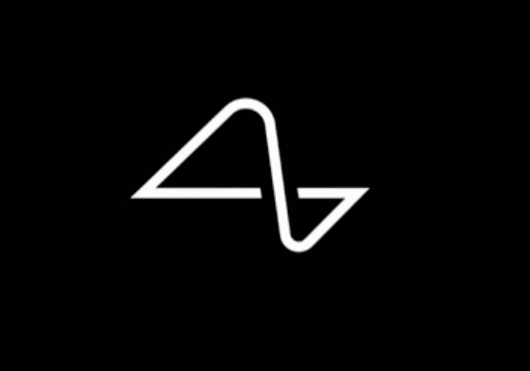
A neuroscientist’s thoughts on Neuralink's announcement
September 2, 2020
One of the most anticipated general public neurotech events of the year happened last Friday: Neuralink’s second yearly update. Although last week was a particularly eventful one for neuroscience, with major announcements from multiple key players in the field, Elon Musk commands a level of attention unlike anyone else (warranted or not). So today we’ll focus on Neuralink and the technology they unveiled.
The Neuralink system
The system Neuralink presented during Friday's event is an evolution of the system they unveiled a year ago. Instead of implanting a single array of rigid electrodes into the brain, as is more common, their system inserts individual threads using a device reminiscent of a sewing machine. Indeed, a significant share of their efforts has been geared towards building a medical robot (i.e. the sewing machine) capable of quickly and precisely inserting the soft and flexible electrode threads into the brain. Individual threads are then connected to a custom chip that filters and compresses the recorded neural data, before sending it out through a low energy Bluetooth connection to a smartphone. A single chip can connect to around fifty to a hundred threads, each with multiple independent contacts, for a total of a thousand individual recording (and stimulation) sites. One of the major changes in the newly unveiled “second generation” device is that the entire system fits inside a capsule about the diameter of a quarter dollar coin, albeit five times thicker (2.3cm in diameter and 0.8cm thick). This package is meant to replace the piece of skull removed during surgery. In other words, a coin-sized hole is drilled into the skull, the electrodes are inserted into the brain using a robotic sewing machine, and then the hole in the bone is plugged with the device rather than the removed piece of skull. This allows for an elegant implant with limited wiring and no transcutaneous connectors. Of note, the surgical robot has also been significantly streamlined and improved, presumably moving it closer to something that could be used in an operating room and on humans. However, details were scarce, making it hard to evaluate any of these changes accurately. The short version is that the technology was very much in line with what had been shown last year, only improved, as can be expected of a company iterating towards a commercial product.
A presentation light on details
After their presentation last year, Neuralink received considerable backlash from the scientific community for excessive hype and failing to recognise similar existing technologies. While hype was still available in spades this year, there was in my view an effort to somewhat scale down ambitions. For example, Neuralink’s mission was stated in much more concrete terms this year: "The purpose of Neuralink is to solve important brain and spine problems." While there had certainly been talk of neurological disorders last year too, they had been overshadowed by very aspirational and futuristic mission statements within the first 5 minutes of the talk, including the distant idea of healthy people using brain interfaces to "achieve a symbiosis with artificial intelligence”. These ideas still made it onto the stage this year, but only later in the event during the speculative part of the Q&A session. Similarly, engineering efforts have been refocused and concretised. Last year’s plan of implanting 4000 channels in their first human participant appears to have been scaled down to a single 1024 channel device. This brings them much closer to the roughly 250 channels currently implanted in human participants enrolled in research studies, including at the University of Pittsburgh where I currently work.
While some neuroscientists are allergic to the hype and the grandiose statements Elon Musk is known for, it’s important to remember that Neuralink isn’t a research lab—it's a Silicon Valley startup. Their event had over a hundred thousand live streamers (despite being the middle of the night in Europe), and the video has since amassed over 2 million views. That’s a big reach—this was no scientific conference talk. A startup’s CEO needs to straddle the line between realistic short term goals and an overarching mesmerising vision to recruit talent and raise money. It’s the same duality that leads to sharing images of towns on Mars (perhaps even with a human-made atmosphere) all while building the world’s most advanced rockets. In Neuralink’s case, there is the added ethical danger of presenting treatments that clearly won't exist for decades, raising false hopes in people afflicted by neurological disorders. However, by offering no explicit timelines or plans, Neuralink is making no promises, only engaging in long term speculation. The same way we can trust people not to sell their homes in preparation for a move to Mars, we should trust them not to take dramatic health decisions based on 20 minutes of sci-fi thought experiments and speculation.
Neuralink’s scientific and engineering merits ought to be judged on the systems it produces, not its storytelling. That is what I will try to do here. Since there is simply not enough information to make a thorough evaluation—educated guesses will have to do.
Neuralink’s strength is not science, it’s engineering
Despite the aforementioned lack of details, a few laudable achievements were on display at Friday's event. The biggest was a demonstration that Neuralink's new device (Neuralink’s Neuralink?) works in vivo (in pigs), and did so for two months. The ability to wirelessly stream from 1024 cortical channels in a freely behaving animal is a big step in the right direction and a demonstration of what money and a talented team can deliver. The same is true of another extremely well-funded neurotechnology startup: Kernel, as I discussed after their last announcement. In both cases, the real breakthrough is the ability to take existing prototypes from research labs and integrate them into a well designed and engineered product. The robot and implantable system Neuralink showcased are more polished than anything currently available, even if none of the individual ideas or components is completely novel. At the end of the day, execution is what matters. The history of technology is littered with examples of companies successfully combining existing ideas and prototypes into compelling products and popularising them (e.g. Apple’s first successful point and click user interface, which borrowed from Xerox's PARC in-house prototypes).
The biggest challenges still lie ahead
While the engineering progress is impressive, where Neuralink still needs to do some convincing is on the science. What we have seen so far is excellent execution of things we knew could be done (miniaturisation, wireless system, surgical robot, etc). The next steps for Neuralink will increasingly involve tackling unsolved scientific challenges, which are an entirely different flavour of problem. Science is much harder to rush, it's less amenable to a startup’s timeline. For instance, Neuralink currently uses thin-film based electrodes, which have yet to demonstrate long term viability in academic labs (where they are extensively studied and have been for some time). Thin films have interesting properties such as very small sizes, high mechanical flexibility, and well-established fabrication processes, which make them well suited for brain implants. But their long term stability remains a looming challenge. So far Neuralink has demonstrated their electrodes can work for 2 months in pigs. That’s a long shot from showing they can work for five or ten years in humans. As Matt Angle, the CEO of another high profile neurotech startup called Paradromics, recently put it: “if you want to test whether something can last 10 years, you really have to wait 10 years.”
The other big challenge is what to do with a thousand channels once they are implanted and stable. Many of the applications mentioned in the presentation are plausible and actively researched (e.g. stimulation of visual cortex to restore sight, stimulation of the somatosensory cortex to restore touch, etc). But each of these is an active area of research full of unanswered questions. Having a thousand channels instead of a few hundred will not significantly change the game. For instance, we know that electrically stimulating any of these sensory areas can elicit corresponding percepts (e.g. localised flashes of light when stimulating the visual cortex). But these sensations are often crude replicas of their natural counterparts, with limited spatial resolution, unnatural qualities and limited dynamic range. While one can extrapolate from these results and imagine a future where improved artificial sensations become practical and useful, it will take a lot more than increasing the number of available channels by one or even two orders of magnitude.
Talking only about stimulation is perhaps a little unfair, since it's the less advanced side of the neural interface coin. Recording information from the brain is much better understood, especially for motor areas, as illustrated by this study from the University of Pittsburgh where a brain implant is used to control a robot with 10 degrees of freedom. Neuralink's first demonstration will likely be to help a paralysed person control a computer with their mind, or perhaps even a robotic device of some kind (this second one is less likely because of the added complexity). Concretely, a system like this could allow the user to interact with a computer by controlling a cursor (i.e. point and click) and input text (either by using a virtual keyboard, or perhaps a more cutting-edge approach like the one I discussed in a recent post). On top of that, the user could control a few additional virtual “buttons”. In other words, the first Neuralink product could be a virtual brain-steered controller, with a joystick, three or four buttons and a speech to text feature. While these are all things that are done routinely in research labs (see for instance Nathan, who is part of a study at the University of Pittsburgh, playing Final Fantasy with his brain implant), providing this functionality in a simple, user-friendly, take-home device with a safe surgical procedure would likely be a useful product for severely paralysed people.
To conclude, Neuralink has integrated some of the best ideas and prototypes from academia into a very polished and elegant cortical implant solution—they’ve built a fine ship, probably the best ship out there (certainly has a lot of sails). But they are now about to embark on a journey through unmapped and treacherous waters. Let's hope they don’t get lost.
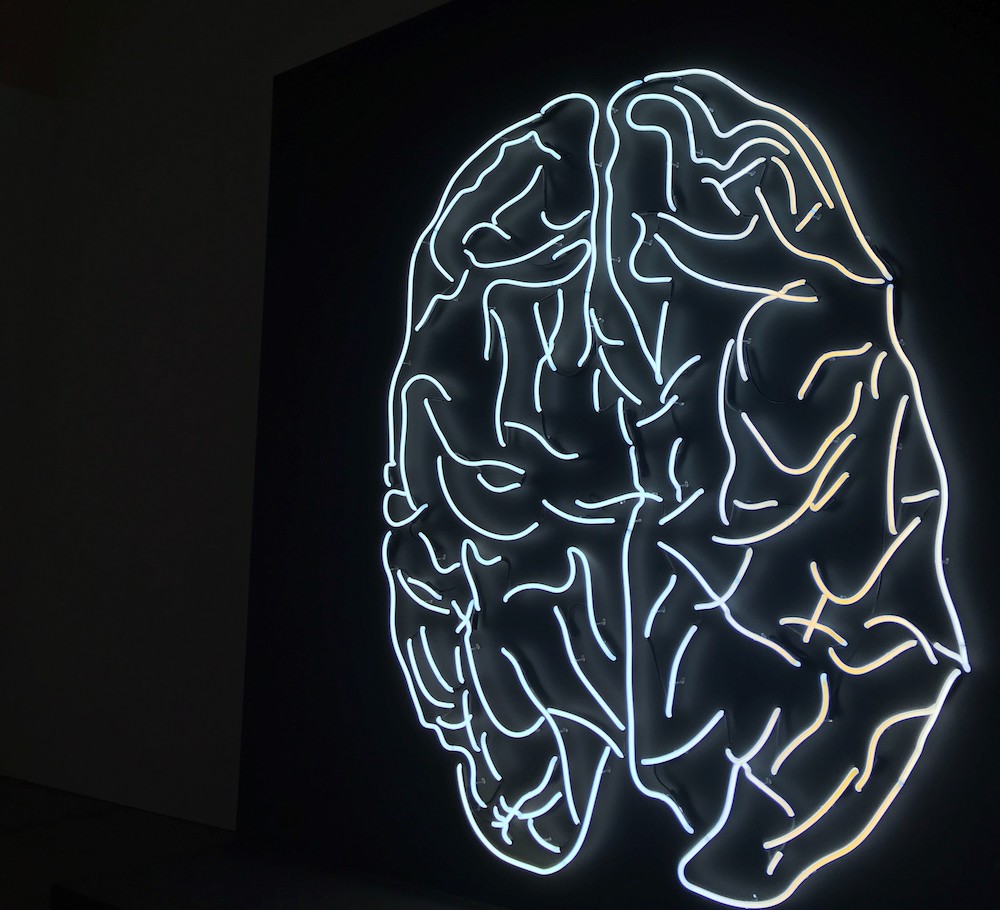
This article originally appeared on Psychology Today
The promises of modern neurotechnology are plentiful, but perhaps none are quite as enticing as the prospect of using brain-computer interfaces for high-speed communication. Efficiently turning thoughts into words could help people with neurological disorders interact more seamlessly with the world around them, and in a more distant future, enable anyone to reach new levels of symbiosis with our increasingly omnipresent digital realities.
Unfortunately, even the most advanced neural implants available today, coupled with state-of-the-art algorithms, cannot reach anywhere near the interaction bandwidth achieved by a healthy pair of hands. A striking example of this shortcoming is text input. An average adult typing on a keyboard produces about 40 words per minute (exact values are hard to come by and depend on a very large number of factors, but this is a fair estimate). On a phone and with two thumbs, people are slightly slower, with an average of 36 words per minute (Palin et al., 2019). Interestingly, younger age groups (i.e. digital natives who have used smartphones from toddlerhood) achieve close to 40 words per minute, comparable to keyboard users.
Far from these speeds, the best invasive brain-computer interfaces to date only achieve about 8 words per minute. There’s clearly a long way to go. While there are multiple reasons for this stark difference, one of them is how typing happens with a brain-computer interface. Typically, a user will move a cursor on a screen (a mind-controlled computer mouse if you will) and use it to click on a virtual keyboard. This is similar to the painfully inefficient experience of entering text on a TV with a remote control: a single letter at a time. One can easily see why this leads to low typing speeds. To be clear, even a slow interface can be beneficial, especially for people with severe motor disabilities who have a hard time communicating or interacting with a digital device. Still, improving communication bandwidth is a major goal for brain-computer interfaces, with far-reaching clinical implications.
A new approach, described in a recently released pre-print article from Prof. Krishna Shenoy’s lab at Stanford, proposes to dramatically improve the experience of brain-controlled writing (Willett et al., 2020). The fundamental idea is simple: What if instead of controlling a cursor to click on virtual keys, one simply imagined writing letters with a pen?
A brain microelectrode array.
To achieve this, the study participant, who suffered a spinal cord injury, had two arrays of electrodes inserted in the area of his brain responsible for moving his right hand. Each of these arrays can be thought of as a “bed of nails” with about a hundred individual shafts (i.e. “nails") penetrating the brain (see picture). The activity of a handful of neurons close to the tip of each of these “nails" can be measured, allowing researchers to eavesdrop on the complex “neural symphony" of this small region of the brain. By listening in on the neural activity while the participant imagined writing letters with a pen for several hours, the authors were able to train a machine-learning algorithm to predict what letter the participant was trying to write based on its “neural signature.” Once the algorithm was trained, it could print letters on the screen almost as soon as the participant imagined writing them.
Using this new approach, the study participant was able to input text at a rate of just under 20 words per minute, about double what was previously possible with this type of brain implant. Importantly, this impressive gain in speed came without any changes to the implant. It was simply the result of a smarter strategy and better algorithms. One of the reasons this new technique performed better is the significantly reduced reliance on precision. Clicking an arbitrarily small virtual key requires accuracy. The smaller the key, the more precise (and slow) the pointing needs to be. But make the keys larger and the distance between them grows, negating much of the gains with increased travel times. Instead, when writing a letter with an (imagined) pen, the entire trajectory matters, not just the endpoint, relaxing the constraints on precision and speed.
While impressive, these results still fall short of average typing speeds attained with keyboards and smartphones, and about 5% of characters were misidentified by the software. This highlights the long road these systems still have to go before they can match physical interfaces.
Nevertheless, that “simple” changes in strategy can improve performance from 8 to 20 words per minute is a very promising sign. It hints at the possibility of using new paradigms to overcome the limited bandwidth of modern brain-computer user interfaces. Given that handwriting is typically limited to about 20 to 30 words per minute (Hardcastle et al. 1991, Burger et al. 2011), it’s likely that future gains in speed will require even more creative solutions, such as using stenography instead of longhand, or abstract interaction schemes that bypass the “physical" constraints of a virtual keyboard or pen.
References
Palin, Kseniia, Anna Maria Feit, Sunjun Kim, Per Ola Kristensson, and Antti Oulasvirta. "How do people type on mobile devices? Observations from a study with 37,000 volunteers." In Proceedings of the 21st International Conference on Human-Computer Interaction with Mobile Devices and Services, pp. 1-12. 2019.
Willett, Francis R., Donald T. Avansino, Leigh R. Hochberg, Jaimie M. Henderson, and Krishna V. Shenoy. "High-performance brain-to-text communication via imagined handwriting." bioRxiv (2020)
Hardcastle, R. A., and C. J. Matthews. "Speed of writing." Journal of the Forensic Science Society 31, no. 1 (1991): 21-29
Burger, Donné Kelly, and Annie McCluskey. "Australian norms for handwriting speed in healthy adults aged 60–99 years." Australian occupational therapy journal 58, no. 5 (2011): 355-363.
Kernel, the neurotech company founded by Bryan Johnson, just released a wave of new information about the technology they have been building over the past half-decade. With this announcement, we finally get a glimpse into the secretive company’s plans. We knew that they had ditched their intentions of pursuing invasive brain recording techniques, leaving that all to Neuralink, a similarly minded effort launched by serial entrepreneur Elon Musk. There were rumours of a NIRS (near-infrared spectroscopy) based system, but nothing concrete. Well here we are, so let’s get to it and unwrap some of the information revealed today.
The system(s)
The vision Kernel outlined in their post is that of a multimodal recording system based on MEG (magnetoencephalography, a mouthful) and NIRS (near-infrared spectroscopy). Both of these technologies are already available and widely used in research settings (and clinical settings too in the case of MEG). What’s new here is the package, miniaturization and ease of use. Far from trivial, these advances could allow both recordings modalities to move out of the lab and into more “ecological” settings (e.g. use during movement, daily activities, etc). If Kernel developed a robust system which successfully deals with the many notoriously tricky drawbacks of both NIRS and MEG, this would be an important contribution to the field, helping democratize these two underused techniques. The general thinking is that MEG offers something similar to EEG (high temporal resolution, limited ability to localize signal), only with better overall signal quality (at least theoretically, we haven’t seen any raw data), while NIRS offers a portable equivalent of fMRI (functional magnetic resonance imaging), at the price of reduced access to deeper brain structures and lower spatial resolution.
Although both modalities were already in use in research laboratories, Kernel’s contribution here is twofold: they tackled some of the key limitations of these techniques, and they produced a more polished and miniaturized version of each. The most exciting advance is the active magnetic shielding in their MEG system which, according to their post, allows them to acquire signals outside of a shielded room (active shielding has been proposed in the past, but not to the point of bringing the system outside shielded rooms, such as here and here). However, the data they report (more on that later) was acquired inside a shielded room ("All experimentation took place inside a magnetically-shielded room to attenuate environmental noise."), which raises the question of how well the system works in practice, and what the hit to signal quality might be in more realistic everyday environments. Still, this would be a big advance, dramatically increasing the scenarios in which MEG could be applied. Another important point is that the MEG system developed by Kernel has a (comparatively) small size. This is possible because they use optically pumped magnetometer (OPM) sensors, which allow for much smaller footprints than the more traditional MEG devices which require extremely low temperatures for their superconducting sensors to work, and therefore very bulky and immobile systems. OPM sensors have been used to build small systems in research settings (see here and here for examples), but nothing quite as polished and with such a high channel count as what Kernel unveiled.
Both the MEG and NIRS systems (which for now are two separate helmets) weigh in at roughly 1.5kg each, which is a lot to be carrying on the head. Having worked at a company intent on placing computers on people’s heads (Magic Leap, which produces glasses that weigh 260g), I can safely say that those weights would not work for any kind of prolonged use and would likely cause musculoskeletal issues in the long run (even the “heavy" Microsoft Hololens comes in at only 566g). Which brings us to the next point: these are obviously not consumer devices meant to be worn for hours on end, or even assistive devices for people with sensorimotor disorders (and to be clear, they don’t claim to be). These are research devices, to be used for neuroscience studies. This raises an important question about a company like Kernel: if their ultimate goal is to pursue general consumer applications, which are far on the horizon, what will sustain them in the shorter term? Kernel may have an answer in “neuroscience as a service."
The compelling (albeit tricky) vision of neuroscience as a service
Neuroscience as a service (coined NaaS by Kernel) is the idea of offering companies the ability to run state-of-the-art neuroscience studies by leveraging Kernel’s in house expertise and technology. The idea is simple and compelling, and one can easily imagine this creating a lot of interest from companies working in UX, learning, rehabilitation and more. Many (if not most) companies do not have the capacity and know-how to perform neuroscience studies to guide their product design and development. Yet many could benefit from these types of studies. Our neuroscientific knowledge may be too limited to learn anything of value in most industries, but it certainly seems plausible that this could work for a subset of companies and use-cases.
Nonetheless, a proposal like this ought to (and certainly will) raise a number of red flags in any neuroscientist’s mind. With our limited knowledge about the brain, and being able to access but a small percentage of ongoing activity with non-invasive sensors, things can quickly veer into pseudoscience. In my view, this is one of the biggest risks Kernel faces with NaaS. It’s easy to envision how a number of companies with little to no interest in rigorous neuroscience may try to add some trendy neurotech spice to their marketing campaigns (I can already hear the taglines: "this is your brain on X”). Luckily, offering this service on their own terms allows Kernel to tackle this issue however they please. But market forces may conspire against them, pressuring them to strike a difficult balance between scientific rigour and profits (i.e. smaller studies will have less power, but will also be cheaper, etc).
A few words about the two demo applications
Together with a description of their system, Kernel also released two demo “studies” serving to establish the company’s scientific and technical prowess. Although somewhat expected of a company trying to generate hype, the wording of their announcements significantly oversells what they achieved in these experiments and how it relates to previous work. Both experiments show nice results and support the quality of their system, but neither of them is groundbreaking. Unlike the headlines, the full-length article is more transparent about the significance of these two studies.
In one experiment, which they call Sound ID, Kernel scientists extended a previously published study. Their headline reads: "Kernel Sound ID decodes your brain activity and within seconds identifies what speech or song you are hearing.” In practice, the experiment works as follows: a person is made to listen to ten predefined and preprocessed song or speech excerpts, and the system will guess which of the ten auditory fragments the person is listening to based on the MEG signal (after tens of seconds). Importantly, this requires knowledge about the piece being listened to. Additionally, this approach would most likely degrade very quickly as one started adding more snippets to it (e.g. recognizing one out of a hundred songs the user might be listening to, etc). This is certainly an interesting illustrative example but is unlikely to be practically useful in this form. Although not from Kernel directly, suggesting this is a “Shazam for the mind” is definitely deep in hype territory. On the other hand, an interesting application of this technique which came up in my research is the ability to detect which auditory stream a person is attending to, for example when multiple people are talking at the same time. It’s easy to envision how this could be helpful in studies on attention as part of Kernel’s NaaS offering.
The second experiment was a more standard speller scenario, where a person can (slowly) type by visually attending letters on a virtual keyboard. This relies on a (widespread) smart trick: each virtual key on the screen flickers with a specific pattern, which is then reflected in the brain when a person looks at it. This way, it’s possible for the system to predict which key the person is looking at by recognizing the flickering pattern. This type of approach works well, and can allow people with motor impairments to communicate (e.g. someone with paralysis after spinal cord injury). What’s intriguing about this choice of experiment is that it highlights one of the main advantages of invasive neural interfaces (of the type Neuralink is pursuing). Specifically, the speller trick requires the person to “type” with their eyes, which is not a very fast and efficient way to enter text (the information transfer rate Kernel found was around 0.9 bit/s). On the other hand, invasive interfaces have been shown to allow speech synthesis directly from neural recordings (see here for another example), potentially enabling a “direct brain Siri” type of interface. Neither of these papers specifically look at information transfer rates, but they should be close to that of natural speech, which some estimates put at 40 bit/s. This highlights the dramatic difference between the two approaches. So while there is nothing wrong with this “speller” demonstration, it’s an odd choice, because it happens to be one of the areas where invasive interfaces have an objective, well-quantified performance advantage over non-invasive alternatives.
Final thoughts
It’s exciting to live in a time where neurotechnology receives so much attention and funding. One of the things extremely well-funded companies can achieve that researchers cannot (and have little incentive to), is building integrated and polished systems. Both the Neuralink and Kernel announcements have that in common: they take state-of-the-art research technologies and turn them into actual products. That’s no small feat and requires a lot of resources. In both cases, the resulting systems are many researcher’s dreams come true, offering signal quality, reliability and ease-of-use well beyond what clunky, semi-custom lab rigs can offer. Although researchers certainly cannot complain, one wonders whether such monumental efforts make financial sense. These companies share a common vision of a future where we interact with our digital tools in more efficient and seamless ways, using our brains directly. But there remains a long and windy road before this vision can become a reality for everyday consumers, and how easily these companies can sustain themselves until that day is still to be seen. Getting there will require more than streamlined and optimized versions of tools researchers already have. The science isn’t quite there yet, and some fundamental breakthroughs will need to happen before many of the promised sci-fi sounding application can see the light of day. The fancy tools Kernel and Neuralink are building will help get us there faster. Here’s to hoping they’ll have the patience to stick around and continue building them while Science meanders along its slow and steady path to the future.
Keeping a daily diary is an oft-touted habit, the benefits of which are promoted as seemingly boundless. Although much of it can easily be dismissed as hyperbole or click-bait, my personal experience in the matter has transformed me from a cautious skeptic into an enthusiastic convert. Here is what I’ve learned after keeping a daily diary for a thousand days (and counting).
It helps build self-accountability
Not being able to keep oneself accountable is arguably the major source of wasted dreams and failed ambitions. It’s what keeps many of us from achieving the goals we set out for ourselves. One of the culprits is the lazy part of our brain (what Tim Urban’s calls the instant gratification monkey), which is constantly trying to jeopardise our plans by pursuing instant gratification. Unfortunately, many of the things the long-term planners in us want are things which require tedious work in the now, and only provide their rewards in the vague then. The only way to achieve those things is to put in the necessary effort. But with a mischievous monkey sitting in our brains, it’s easy to just give up, procrastinate, just this one time, just for today. With no deadlines, no accountability, there really aren't any negative consequences for skimping out on the hard parts. It’s only looking back that one may regret not achieving the things they had set out to do, and by then it may be too late.
That’s where the diary comes in. It's certainly not perfect, and it certainly won’t defeat the "procrastination monkey" on its own, but it’s a start. The reason it helps is that by sitting down and writing about your day, you are helping keep yourself accountable. You are effectively forcing yourself to stop and think for a moment, to put things into perspective, evaluate them in retrospect. If you’ve been using your time in ways you had not planned to, keeping a diary helps you realise you've been cheating yourself. To put it simply, without a diary, pursuing instant gratification may not lead to anything bad — life just goes on and your temporary qualms get brushed aside. But with a diary you get to disappoint yourself again and again, every day. This pleasant exercise forces you to face the truth and hopefully gives you the drive to look for solutions.
It’s a great tool to shoe-horn other habits into
Keeping a diary every day is a habit one needs to develop, and like most (good) habits, getting it to become second-nature takes time and discipline. There’s a high chance that you will stop early on, when it’s hardest to keep going (like those gym memberships that just sit there from February onwards). But if you successfully reach the point where daily logs are simply part of your life, congratulations, you’ve unlocked a very powerful tool for self-improvement.
The reason it’s such a powerful tool is simple. It’s easy to use an established daily diary routine to boost other would-be habits. For instance, if you wanted to work on your novel daily (say, by writing a thousand words), you could simply add a “novel word counter” entry to your diary where you record how many words you managed to put down every day. By doing so, you are using your existing, established routine to force yourself to think about your other goals every day. It’s easier to build a habit on top of an existing habit than it is to build a habit from scratch.
You might just get to know yourself a little better (and fight back your inner biases)
What you write in your diary will depend on what you plan to use it for. But whatever you do write, it’s likely to involve crystallising some of the thoughts running around your mind into concrete written sentences. The simple step of forcing yourself to put words onto your thoughts helps you understand them better. This is the well known power of introspection.
Behavioural neuroscience has established we are excellent "confabulators", making up stories to justify our actions and thoughts. We are also essentially blind to the world around us, experiencing only a small part of it, and making up the rest. In other words, we paint a picture of the world tainted by our specific biases and beliefs. Considering these limitations, it’s easy to see how a decision we took a while back might suddenly seem like a strange move under a new light (or simply in a different state of mind). The power granted by a diary to read through your own thought processes provides invaluable insight into your own beliefs, biases and thought patterns.
Of course, a diary is no magic wand. It may help shed some light on your mind’s shortcomings. Overcoming them, however, requires intellectual honesty and a healthy dose of self-criticism, assuming it can be done at all. The existence of (and fight against) our inner biases is something Bryan Johnson explores in great detail in his blog post, which is worth a read.
Offloading memories and creating traceability
Finally, a more tangible and practical benefit of keeping a daily diary is the fact that anything you write down will be searchable (if your diary is in digital form) and available for future reference. Depending on the level of detail that goes into your daily scribbles, you diary could help you remember anything from important events to mundane details. Remembering when you took that holiday to the sea may only be good for proving your spouse wrong (disclaimer: I do not recommend ever proving your spouse wrong), but knowing what your boss told you about that project which seemed irrelevant at the time but is now crucial to your career could be a life-saver.
We established that our brains are full of biases and don’t really see the world the way it is. The same hold true for our memories. They are a mere shadow of past events, deformed by time, filtered by our perception and emotions. Having a reliable account of important events is an objectively useful aspect of keeping a diary, and comes in handy more often than one might assume.
Keeping a daily diary may not be the most ambitious goal you decide to pursue, but it might just end up impacting your life in more ways than you anticipated. It’s a low investment high return kind of deal. So get writing.
A few days ago, Elon Musk’s latest venture was finally revealed after months of dropped hints and speculation. The new company, Neuralink, will presumably pursue the futuristic sounding goal of intelligence amplification (IA), joining Kernel, a slightly older effort with analogous goals launched by Bryan Johnson. Practically, these companies will work on developing new techniques to link the human brain to computers. Although many such brain-machine interfaces (BMIs or BCIs) exist today, none of the current systems are capable of precise, large scale recording of the entire brain over long periods of time, while at the same time minimising the risks associated with the initial insertion procedure (ideally forgoing the need to crack the skull open). Clearly, in order to take any meaningful steps towards intelligence amplification, both companies will initially have to focus their efforts on creating a new neural interface, one allowing for a high-bandwidth communication channel between brains and computers.
Fittingly, these efforts arrive at a time where the field of brain-computer interfaces is ripe for momentous achievements. In recent years, following the clinical success of implantable BCI systems in humans (great examples can be found here and here), a multitude of new and promising designs for neural interfaces have been proposed. These novel designs aim to overcome the technical challenges of current generation systems, such as deleterious immune responses, tissue damage and chronic stability issues. Kernel and Neuralink are likely to be pursuing ideas which are extensions of the current state-of-the-art, possibly working on several promising approaches in parallel. Some recent and intriguing developments include: stentrodes, small electrodes inserted through the veins, making for a minimally invasive insertion procedure, brain dust, tiny wireless electrodes designed to be sprinkled throughout the brain and powered externally via ultrasound, and injectable mesh electrodes, nano-scale polymer meshes which are injected with a syringe and then unfolded in place. Considering Elon Musk’s fondness for the sci-fi term neural lace, this last approach may be what Neuralink will focus on at first.
Of course, advances in such fundamental areas as brain-computer interfaces will have far reaching implications, extending well beyond the somewhat distant goal of intelligence amplification pursued by both ventures. Indeed, if they succeed in obtaining even modest gains in the pursuit of improved neural interfaces, they will find willing collaboration partners in areas such as brain disorders treatment, fundamental brain research, control of robotic devices for paralysed patients, and many more. Although their intelligence amplification vision may be lofty and unattainable in the short term (Elon Musk mentioned a timeframe of 5 years, which is somewhere between optimistic and insane, depending what one defines as "meaningful intelligence amplification”), the immediate clinical benefits will be significant. In fact, these efforts are likely to play out similarly to the way SpaceX did—first delivering short term benefits which also happen to be financially viable (e.g. commercial rockets to deliver satellites), before eventually attaining the real goal (in the case of SpaceX, trips to Mars and beyond). Despite having directly applicable benefits, focusing communication efforts around the long term vision is smart and necessary. The hype fuels massive non-technical followings on the internet and beyond, while at the same time, articulating a well reasoned long term mission helps recruit the top minds in the field who share the same vision.
Speaking of long term vision, as Elon Musk dramatically puts it, in this case the stakes are extremely high (existential-threat-level high). With the advent of general-purpose AI around the corner (again, “around the corner” could mean anything between 5 to 100 years depending on whom you ask), the risks associated with super intelligent computers are looming ever more menacingly in the background. Beyond making entire job segments obsolete, what many are afraid of is the immeasurable harm a powerful AI could do in the wrong hands, along with the not-so-unlikely prospect that somebody may trigger an apocalypse scale disaster by mistake (Elon Musk thinks Google is
a good candidate for the biggest “oops” moment in humankind). To mitigate the dangerous aspects of AI, the approach both companies are working on (and which I have proposed in the past) is to ensure that by the time we have the technology to build super intelligent AIs, we will also have the technology to seamlessly interface our brains with computers. That way, any advance in AI technology can presumably be used to benefit human intelligence directly, making it hard for AI to become independently more advanced than human intelligence. Problem solved.
As some have been quick to point out, however, it may not be as simple as that. Indeed, one could argue that the same risks associated with super intelligent AIs could be equally problematic in the case of super intelligent humans. There is no guarantee that a super intelligent person, whose motivations, thoughts and desires we cannot begin to imagine (by definition), will not decide to act in a way that is dangerous or harmful to “lesser" humans. In fact, one could articulate plenty of scenarios where intelligence amplification leads to non-desirable futures, such as hyper-segmented societies where the rich can afford to become super intelligent while the poor are stuck with stupid old human brains, leading to a widening economic divide and increasing social tensions.
Still, all this is speculation. The only certainty is that if Neuralink and Kernel succeed, the world will benefit immensely in the short-term, especially when it comes to understanding and treating our ageing brains, a challenge we will increasingly face as humans grows older. Will these companies save us from an AI apocalypse which might never come to pass in the first place? Probably yes, in fact, but only time will tell.
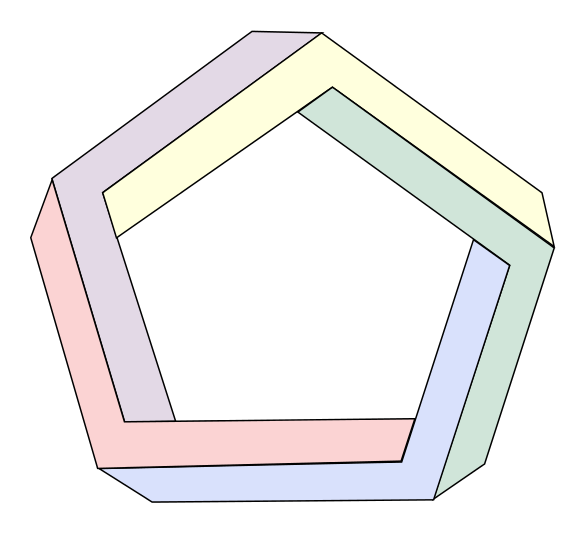
In the wake of the US presidential election, an important issue has been brought up: social media is full of fake news. Some go so far as to suggest this may have significantly swayed the final result. Regardless of the answer to that question, the issue of false claims on the internet is a very profound one, and reaches much, much further than the realm of politics. There should be no sugar-coating it: misinformation is dangerous, and a potential threat to society.
As the debate rages on, some of the large internet companies (notably Google and Facebook) have chimed in, offering solutions with varying amounts of commitment. The specifics remain unclear, but there is a general notion that stories should be fact-checked. Based on this information, one supposes, fake news would be labelled, or perhaps even removed. I believe this is the wrong way to address the issue. Here’s why:
1. False facts are dangerous, but partial information is worse
The problem with fake news is unfortunately more complex than presenting wrong facts. A much more effective (and more common) approach is to try and steer people to a certain conclusion by giving them only some of the facts. Let’s take the following example: “Human exposure to X-rays can lead to severe burns, cancer and even death. X-rays are classified as a carcinogen by the World Health Organisation. Yet, every day, thousands of people are exposed to X-rays in hospitals across the world, including children and elderly people, two groups known to have weaker immune systems.”
Should a fact-checking system flag the above statement as false? Certainly not. None of that is factually incorrect. However, it’s clearly a misleading paragraph. This is achieved by leaving out the following piece of information: the doses of X-rays used in hospitals are very low, so low that any of those scary side-effects are extremely unlikely to occur. Humans are exposed to X-rays from natural sources every day at doses not much lower than this. This makes it easy to see why removing false facts is simply not enough.
I specifically avoided using an example from this year’s election (by fear of sparking an irrelevant debate), but one needs only to open a newspaper and pay attention to see examples of this strategy being used every single day (wether purposefully or not).
2. In the real world, information is often more nuanced than right or wrong
A smart engineer from Facebook might object to the first point by saying that a sophisticated enough system could handle the example above too. It might prompt the reader with a warning: “this information is misleading because it leaves out this and that crucial information.” But this would still be wrong, because it would be pushing the reader towards a specific conclusion, therefore introducing bias. The problem here, is that the paragraph about X-rays and the conclusion it leads to cannot be labelled right or wrong. Wether or not you think the exposure of children to X-rays is acceptable considering the minimal risks, is purely subjective. Any system steering you towards one or the other conclusion is a biased system.
Certainly, you may say, some facts are not nuanced. Some facts are simply right or wrong. And to some extent that is true. For instance, Steve Jobs died on October 5th, 2011. Any article claiming otherwise would most certainly be making a false claim. But as I hope to have shown above, the danger of misinformation doesn’t lie in such openly wrong statements (although that certainly constitutes one aspect of it). The real danger of bad news lies in other forms of manipulation which are much more subtle (such as using partial information). A program which identifies simple false claims will most likely miss these, ironically giving them more credibility by not discrediting them.
3. Facts are not dogma; they should be easy to challenge
Nothing is absolute. Every single fact can be challenged, questioned and potentially changed. That is the foundation of our modern world (and science), based on the enlightenment movement of the 18th century. The very idea of developing a central authority with the power to label facts as true or false, regardless of the nobility of its intentions, is a dangerous step backwards. Of course the aim will be good. But the results may be unintended. Facts are challenged all the time. Will having a system that labels every challenge to an established fact as “fake” result in an increased barrier to healthy debate? Who gets to decide which facts are right and which are wrong? And how do we decide when there is enough evidence to retire an outdated fact?
What we should do instead
The notion that we must remove or hide information is fundamentally flawed. People sharing fake news is a symptom, not the issue. Treating the symptom does little to eradicate the underlying problem. The real problem here is that the average reader doesn’t approach news critically (see my article on 7 ways to think more critically for more on this). Perhaps this is caused by a failure of our educational systems to prepare students for the age of information. Regardless of the cause, the issue is present and must be fought.
Certainly we cannot solve the issue by taking facts away. Instead, we should give more. We should provide tools to help develop critical thinking and approach facts with a more scientific mind. Imagine this: a box after each link (on Facebook, or Google, or anywhere else), which shows two columns. One columns says “here are other articles supporting this view", and the other says "here are articles that view this issue differently". Under both columns, a list of articles, their source and the total number of items in each category would be displayed. No special treatment, no stars next to reliable sources (what is a reliable source anyway?). Equal footing. No bias. We give as many tools as possible, make it as easy as we can for readers to come to their own critical conclusions. Nothing more.
Of course this "critical thinking" box could have many other features, such as an information tab which explains what the source of a given fact is, and the methodology (for instance if a fact was obtained through a census, etc). There is one caveat to this magical box sitting below links. It would be very hard to build. Even harder to build well. But the general idea, which is to help users reach their own informed opinion, is a powerful one, and one that big companies can start pursuing today.
It may be embarrassingly cliche, but the age-old adage fits the situation perfectly: give a man a fish and he will eat for a day, but teach a man to fish and he will never go hungry again. We must provide social media users with the tools for critical thinking, not feed them what we believe is right. The benefits of solving the underlying issue, as opposed to just the symptom, is that people will learn to spot dubious claims well beyond Facebook and Google. They will spot them on the radio, at dinner parties and during political speeches. That makes for a better world.
My plea to social media giants is simple: remember that the internet has always been about access to information, not the opposite. Let's solve the problem with more, not less. If not from me, then take it from Benjamin Franklin: "Printers are educated in the Belief that when Men differ in Opinion, both Sides ought equally to have the Advantage of being heard by the Public; and that when Truth and Error have fair Play, the former is always an overmatch for the latter."
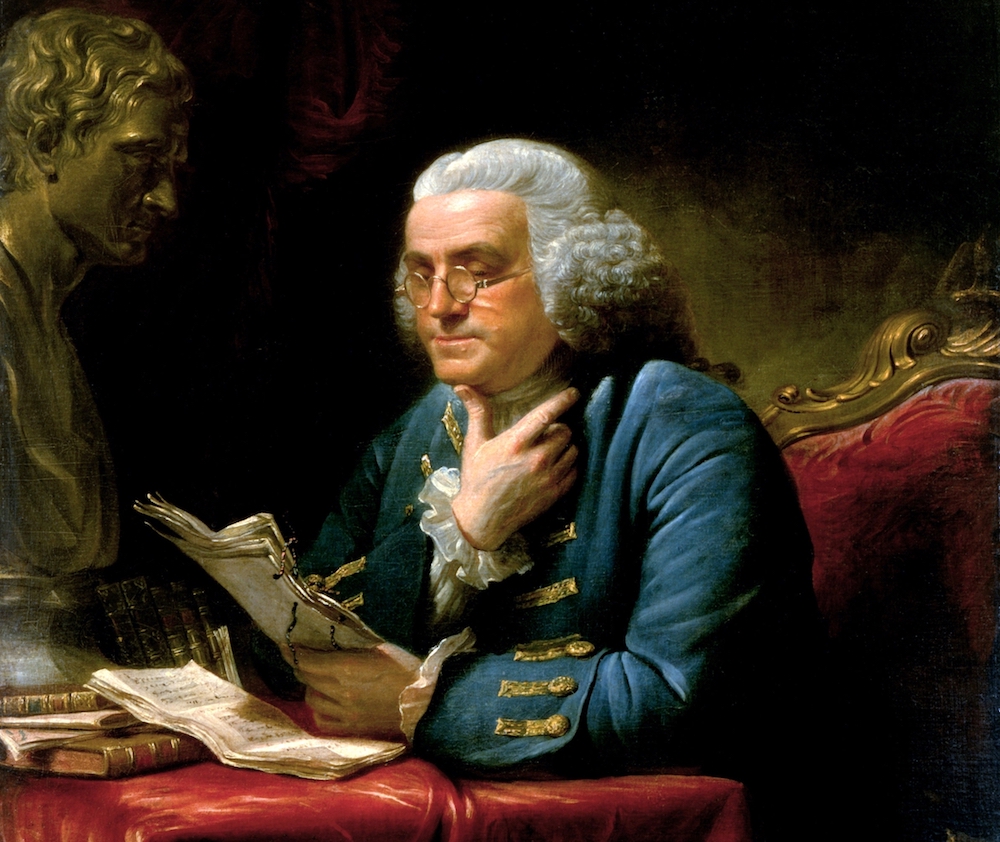
7 ways to think more like a scientist
October 30, 2016
In today’s uber-connected world of hand-held internet gizmos, not a minute goes by without our brains overdosing on new information. Often throughout the day, we are presented with novel ideas, which we must instantly process and store—salt isn’t what’s killing us, sugar is; we’ll get to mars in fifteen years; we’ll never get to mars in fifteen years; GMOs are bad; GMOs are good. And the list goes on and on. Faced with such a constant stream of (often contradictory) data, our minds must necessarily answer the question: what should I believe?
That simple questions hides layers and layers of far-reaching complexity. That simple question controls nations and the fates of entire species, even entire ecosystems—by driving popular votes and consumer habits. If a majority of people think that global warming is fake, they will elect leaders who think likewise and will refrain from halting its progress.
Clearly then, there is tremendous power in our collective decisions to trust one source rather than another, to believe one fact above its alternative. And the question inevitably becomes: how can each of us guide this process to make sure we arrive at the right conclusions?
By thinking more like scientists. And these are 7 ways to do precisely that.
1. Question everything, accept nothing
If you remember only one point from this article, let it be this one. One of the most important traits which allows a scientist to keep things straight is an almost pathological skepticism, a distrust for new facts.
People will say all kinds of things for all sorts of reasons. Even scientists. Some lie, some are nuts and many are simply genuinely mistaken. In other words, everyone is potentially unreliable. That is why no single source should be trusted.
Science and scientific thinking are extremely robust precisely because they do not depend on a central authority. They are scalable and personal—anyone can apply them. A scientist will not take things for granted, regardless of the source. Instead, he will use the steps outlined below to arrive at his own conclusions.
So the next time someone tells you “but, scientists say…”, just walk away—you know better.
2. No really, question everything
There is no emphasising this enough. Regardless of who said it, regardless where you read it: question it. Articles published in the most prestigious scientific journals get disproven or retracted all the time. Even Nobel prizes have occasionally been attributed to discoveries later shown to be highly questionable.
And be careful, your own ideas aren’t any different. They’re not special. In fact, before you start questioning everything around you, make sure you took the time to stop for a while and question everything you know and believe.
3. Check sources
If you feel like this whole critical thinking things will take a lot of effort, your are right. But there are ways to alleviate the burden. The very first thing you should do when reading an article about a scientific discovery, big or small, is to check the sources.
In science, every new piece of research is published in so called peer-reviewed journals. These journals, unlike traditional ones, have an extra verification step before they publish anything. When you submit your work, these types of journals will send your article to two or more experts from the field, who will scrutinise it, looking for inconsistencies and mistakes. Eventually, they may green-light the article for publication. Although far from an infallible system, it serves as a filter—a first line of defence against bullshit.
Of course, blindly accepting things you read in peer-reviewed journals would be breaking the first rule. Instead, you should use these journals as a guide. If a scientist does not publish his results in a peer-reviewed journal, be extra careful, something fishy is going on. A scientist who avoids a peer-reviewed journal is like a salesman trying to auction goods from the back of a shady van—not a good sign.
4. Seek out confirmation
If you can’t trust any single source, then how can you ever accept new science?
The answer is replication. If it’s sound, then others can (and will) arrive at the same conclusion. That’s how science is built. If someone publishes something new and unexpected, other experts will first raise an eyebrow. Then, they will stroll back to the lab, the new article in their hand, sit down and attempt to replicate the results.
Of course, individuals are not expected to replicate results themselves. I’m not saying you should build a particle accelerator in your basement and start searching for signs of a Higgs Boson. Instead, you should wait for confirmation from third parties
In practical terms, unless you are an active scientist in a given field, your safest bet is to rely on review articles, which are also peer-reviewed and usually offer balanced summaries of recent research (with extensive references, for the brave). And if you’re not into reading scientific articles (an understandable objection), make sure the journals you get your news from put in the effort to cite relevant findings, and that those references make sense.
5. And seek out counter-arguments
There is more than on side to any issue. When you read about a position (GMOs are harmful, global warming is a hoax, coffee is good for your health), make sure you look up what the other side is saying. This is an extension of the question everything mantra. By opening your mind to opposing views, you increase your chances of not missing something important. And if you have the tools to critically and objectively evaluate what both sides are saying, you will reach the right conclusion.
6. Learn to spot borderline statistical claims
These last two tips are more practical and less general. They should help you spot some of the most common symptoms of bad science.
Statistics is a vast and challenging field, which many scientists, who are trained in a different topic, grapple with. Without delving into the intricacies and maths, there is one major principle, which once understood, could prevent a large number of misleading claims: correlation does not imply causation.
That simply means that if two things seem to be correlated—if they seem to change together—it does not mean that one caused the other. Let’s take an example. If data shows that areas with high homelessness have high crime—correlation—you may jump to the conclusion that the homeless commit crimes—causation. This would be a misleading statement, because the data does not show that. This type of wrongful conclusion is particularly misleading when the causation is something you might somehow expect (often because of some form of prior bias). In this example, perhaps a third factor, for instance unemployment, causes both homelessness and high crime rates.
This is the single most pervasive error out there. You can open any major publication, on any given day, and are almost guaranteed to find an article which makes that mistake. If you learn to spot it, you will avoid countless misguided conclusions.
7. Don’t be fooled by bad starting postulates
In science, we often build a line of argumentation starting with a few basic postulates, or hypotheses, from which we logically draw other conclusion. For instance in math, some basic rules (or axioms) are enough to deduct countless other rules by pure logic.
However, there is a trick that is often used to arrive at flawed conclusions (knowingly or not), which is to start with a false postulate. Since everything flows logically from the starting hypothesis, this particular error is hard to spot. In fact, you can build an entire fallacy logically starting from a false statement.
I recently saw a video highlighting this precise problem. In the video, a professor argued as follows: humans shouldn’t eat meat because they are not carnivores. He then went on demonstrating in countless ways how humans are not carnivores (they have long digestive tubes, blunt teeth, etc). Although his arguments were correct, the starting premise was false, and thus the conclusion too. Of course it’s easy to find example of why humans are not carnivores, because they are not: humans are omnivores, eating both plants and meat. The initial statement that: “humans shouldn’t eat meat because they are not carnivores” is flawed because carnivores are not the only types of animals that eat meat.
Arguing against this professor’s position would have been very difficult, because he would have asked you to prove why humans are carnivores, an impossible feat. Instead, the trick was that the very premise of his entire reasoning, that only carnivores eat meat, was wrong.
This type of logical construction—starting from a false premise—is very common, and can be very misleading because all the arguments presented after then initial claim are correct and logical. We thus find ourselves agreeing with everything being said, and may ultimately mistakenly accept the conclusion. Remember to always search for the starting premise and see if it makes sense.
I hope these 7 tips will help guide your critical thinking in the future. And remember: science is ever-changing. No matter what conclusions you may draw today, be prepared to change them tomorrow. As someone once said: “science doesn’t know everything? Of course it doesn't, otherwise it would stop!”
Near the end of his book Sapiens: A Brief History of Humankind (an interesting read, if you haven’t had the chance), Yuval Noah Harari briefly explores some options for the future of human evolution. He narrows it down to three scenarios, which do indeed encompass the major foreseeable alternatives. Specifically, he mentions the bionic, genetic and non-organic options. More than mere buzzwords, these three concepts are used to describe entire fields of human technological progress. To put it briefly, a bionic future would be one where humans merge with machines (think of robotic arms and chips in the brain), improving our bodies beyond biological limitations. A genetic future is one were humans are enhanced through the use of genetic engineering, endowing themselves with features worthy of gods (longer lives, sharper senses, and more). And the non-organic future, well, that one isn’t quite as nice for us. In fact, it has humans completely out of the picture—instead, new life forms (robots, software) learn to replicate and spread independently of their creators.
These three options could be seen as mutually exclusive—forces acting against each other, with the winner determining what the future will look like. In reality, I would argue that all three are components of a larger, cohesive narrative, one that has humans soaring high above all other life, breaking free from the shackles of natural evolution, colonising the solar system and why not the universe too, while we’re at it. So let’s take a look at why genetics, bionics and artificial intelligence are the three indispensable wheels in the great tricycle of human progress. That doesn’t sound very impressive, I know—but a tricycle is all we get.
All three areas are currently being pursued actively by researcher all over the world. Genetic engineering is undergoing a public image boom as advances such as CRISPR grab headlines across the world (you can read more about CRISPR here). Bionics and neuroprosthetics are similarly stroking the public's imagination with robotic hands that feel, and other futuristic inventions. And of course, artificial intelligence, and evolutionary algorithms (algorithms that can change over time, inspired by Darwinian evolution) are widely discussed and have far reaching implications, way beyond asking Siri to set a timer (read this for a take on the intelligence behind artificial intelligence).
Is any of these fields going to mature way before the others? Wouldn’t the premature success of one of these scenarios hinder the development of the others? These are extremely interesting questions. In fact, if scientist and engineers actually spoke about such things at lunch, I’m sure these enquiries would take center stage (center table?). But since they do not, the task befalls to us.
Artificial intelligence is among the chief components of the non-organic future. Because it does not involve humans directly in a medical sense and is not technically impacted by the laws of physics, this approach is likely to evolve faster than the other two. But that does not mean it's game over—the future will not automatically be non-organic. All of these technologies should not be seen as binary—artificial intelligence is not an all-or-nothing proposition, nor are bionics and genetics. Each of these futures will start happening progressively and in parallel. In fact all three have already begun. The advent of pervasive artificial intelligence does not preclude advances in genetics or bionics, if anything it encourages them. Each will provide only modest benefits at first, in the image of Siri for artificial intelligence, current (limited) robotic limbs for bionics and the first simple gene therapies for genetics. Then, as these technologies progress, their usefulness will likewise increase. Self driving cars, new cures for cancer and impressively life-like prosthetics—these are all around the corner of progress, along with much, much more.
I have argued that the only way we can avoid being overtaken and possibly marginalised by future super intelligent machines is by merging with them (you can read the article here). Thankfully, the power of bionics will enable us to interface our minds with computers. Genetics will initially play a supportive role, allowing our organic shells (aka bodies) to withstand the test of time longer. However, further into the future, genetics (with the help of synthetic biology) will allow us to develop organic bionics: seamless interfaces between the living and the artificial—where the very distinction between the two starts breaking down. Eventually, organic computer circuits and humans engineered to better interface themselves with machines will blur all lines.
Clearly then, these three driving forces for the future do not act against each other. In fact, they slowly converge to bring us towards a single goal: the human of the future. By taking advantage of the strengths of machines, and combining them with our own genetically improved bodies, we will create a new form of super humans, or Homo Deus, as Yuval Noah Harari likes to playfully call them. These future beings will be so vastly superior to us in every way, that we could not even begin to imagine what their dreams, thoughts and actions will be like.
Genetics, bionics and artificial intelligence—that is precisely how we will make the future.
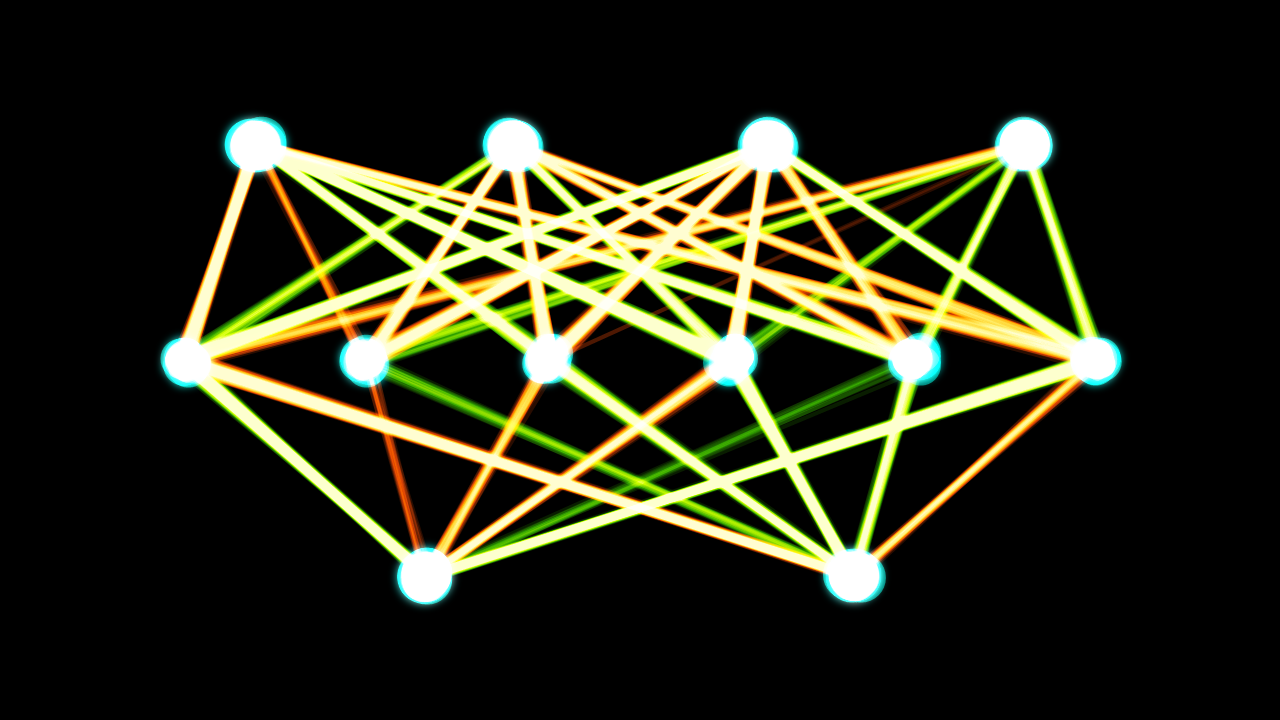
Is artificial intelligence intelligent at all?
September 25, 2016
The world of artificial intelligence (AI) went into a frenzy when AlphaGo, a computer algorithm developed by Google, beat the world’s top Go player. This was remarkable for two reasons. Not only is Go a much harder game for computers to play than chess (which computers mastered almost 20 years ago), but experts in the field were expecting this impressive step to happen as much as ten years later than it did.
Deservedly, the world’s media outlets took this opportunity to re-ignite the debate about artificial intelligence and how it may affect our socio-economical order in the coming years. One particularly important topic in this debate is the notion that improved artificial intelligence could eventually take over many people's jobs. Which is either a terrifying prospect, or an immense opportunity for humankind, depending on whom you ask.
When we imagine computer algorithms taking over jobs and tasks usually performed by humans, most of us get a queasy feeling. People tend to fall into two diametrically opposed categories. The first are those who think computer algorithms could never replace humans (except perhaps in some very simple and repetitive jobs), the second are the tech enthusiasts and futurists who believe that the inevitable progress of science will deliver increasingly complex machines which will not only replace us, but eventually surpass us too (this is called the singularity, which I explore in more detail in this article).
The first group are the AI skeptics. Usually this camp argues that machines cannot make the nuanced, adaptive responses humans can—because they must follow a strict set of rules, and these rules could never account for every possible scenario. Unfortunately this line of reasoning is flawed, because it misunderstands the way modern machine learning algorithms work—relying instead on a more traditional view of computer software, where everything happens according to a set of logical decisions and relationships defined by the programmer (if this happens, then do that). But this is wrong. The whole field of artificial intelligence is based on a very different approach. Indeed, machine learning algorithms are designed to learn how to generalise. The basic idea is as follows:
a machine learning algorithm is shown some examples of data (e.g. a photo recognition software may be shown 10 different images of cars, 10 images of cows, etc). From this data (called a training set), the software learns to extrapolate and generalise. Once trained, the algorithm will be able to understand new data (in our example, it will be able to say if a photo contains a car or a cow, even if it has never seen the photo before). This is important, because it means the algorithm was able to learn rules by itself. No programmer had to say: "if it has four wheels, then it is a car” or other arbitrary conditions. Instead, the software extracted some characteristics from the initial data it was shown, and created its own internal representation of a car. In fact, it is often very hard to peer back inside the algorithm and understand how it takes decisions.
Clearly, this is not the kind of simple rule-following-machine we are used to dealing with. Once we realise how these programs work, we start to see why they are called artificially intelligent. In many ways, they mimic the learning process of a child, who accumulates experiences and uses them to extrapolate and approach novel situations with ease. And because AI learns how do to things on itself, we won’t need to understand how our brain solves some complex tasks in order to teach them to machines—we’ll simply let them figure it out.
The second group are the AI enthusiasts. This camp tends to exaggerate in the other direction—placing an overly optimistic amount of hope in artificial intelligence’s ability to overcome all challenges. Although in the long term this group will eventually be right, the reality is that for the moment artificial intelligence is still very limited. Despite AI’s ability to generalise and adapt to novel situations, for now this is confined to a single subject at any given time. The image recognition software which can recognise cars and cows in photographs would be completely unable to play Go for instance. Training a single algorithm to excel at several heterogeneous tasks is the real challenge, and one which may not be easy to solve in the short term.
Although artificial intelligence programs are certainly smarter than what the AI skeptics would think, they are still a lot more stupid than the AI enthusiast like to believe. We may call them intelligent, but in reality they are based on simple statistics and basic networks of interconnected processing nodes (neural networks). Once we understand how these algorithms work, they lose some of their mystique. It’s the same feeling one gets when an impressive card trick is revealed—the magic is simply gone.
But here is something to think about: perhaps the fact that relatively simple computer algorithms can replicate some complex human behaviours is an indication that our brains may be simpler than we think. Deep down, the reason the notion of AI replacing people is so hard to swallow is the human fallacy that our intelligence is somehow the consequence of some deeper, perhaps mystical force, and that a “simple" machine could not possibly replicate the wide range of behaviours and flexible decision making humans demonstrate. Well, we’re in for a humbling surprise—not only will AI surpass humans in almost every possible occupation, it will also demonstrate that intelligence isn’t as unique and special as we thought.
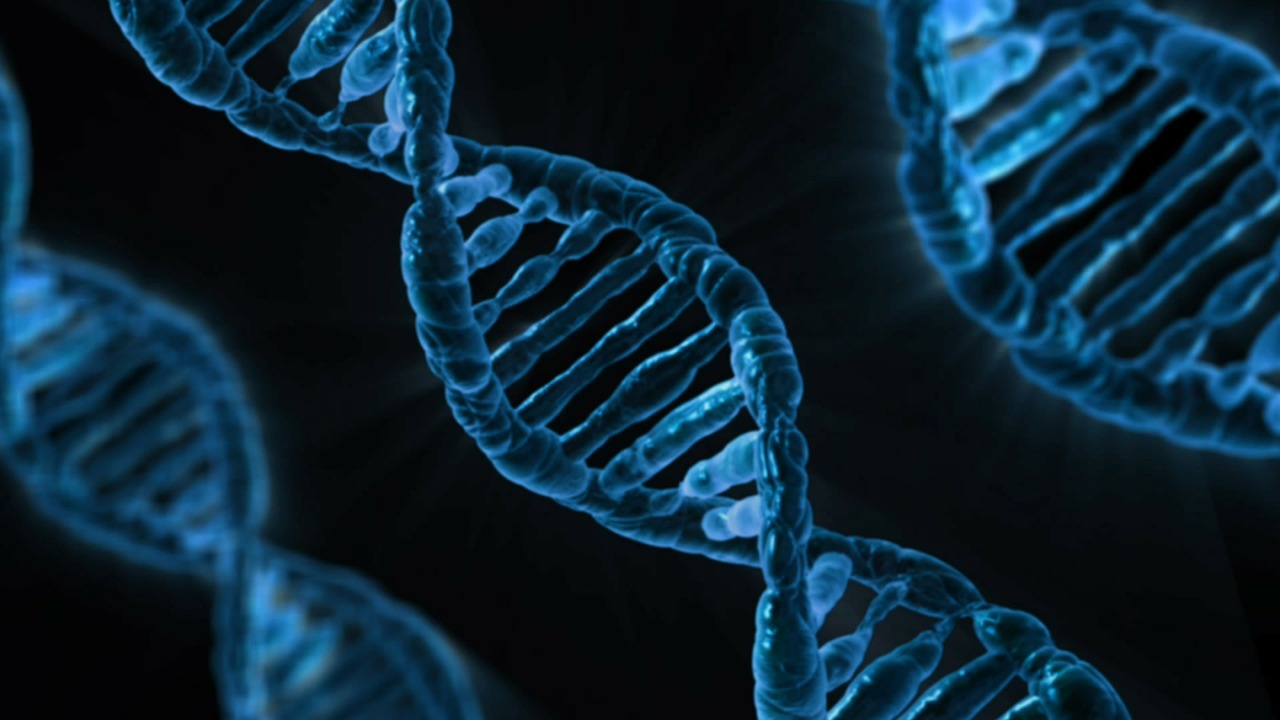
What is CRISPR, how does it work and what does it mean for our future?
September 13, 2016
Do you constantly hear CRISPR mentioned in the news and around the web, but never truly took the time to really understand what it is and how it works? If so, this (relatively) brief primer is just the thing for you. More than just an unpronounceable acronym, CRISPR is a revolution in the making. Considering the impact this technology will have on our future, it’s well worth understanding what everyone is talking about.
CRISPR (a short-hand used to refer to the CRISPR/Cas9 system) is a novel tool for genetic engineering, the manipulation of DNA in living organisms. Although CRISPR was only mastered in 2013, progress has been swift, and some ethical questions that seemed like distant thought experiments are becoming pressing issue we need to address. Unfortunately, as with most great science, a lot of misinformation and sensational headlines often accompany the scientific breakthroughs. That is why the best way to approach this debate—like any debate—is informed.
How does CRISPR actually work?
First, you should know that CRISPR, originally discovered as part of a naturally occurring immune system found in bacteria, is a cellular mechanism which can cut DNA strands (DNA can be visualised like a long string composed of 4 types of sub-units, which together form a language, not unlike the 0s and 1s used in computers). The way this works is based on two components, a pair of scissors and a template. The template is a short segment of RNA (which can be imagined as a photocopy of a short piece of DNA), which is kept floating around in the organism. The scissors are a protein (called Cas9), which can use these templates to identify a specific part of a string of DNA. Whenever the template matches a segment of DNA, it tells the Cas9 protein to cut the strand at the location it specified.
The real breakthrough was the realisation that this system can easily be hacked by using some Cas9 proteins (the scissors) and a custom designed template (it turns our RNA is something biologists already know how to make). Now, whenever the custom sequence defined in the template is encountered, the Cas9 protein will cut the DNA at the specified location. By defining the right template sequence, it is possible to cut anywhere, at will.
Cas 9: not quite the scissors you had in school.
Of course, there are many subtleties, such as inserting a new piece of DNA where the cut was made. To do that, scientists rely on a naturally occurring system, which detects breaks in DNA and repairs them. By taking advantage of this process, it is possible to “trick” the cell into incorporating an additional piece of DNA while it repairs the cut. However, this is harder to achieve than simple cuts, and is currently posing some challenges. This is why the first set of applications will orbit around removing segments of DNA rather than adding new ones.
Although there are many variations, the underlying mechanisms is always the same—diverting the CRISPR/Cas system to achieve one’s goal.
Okay, but back up a little, why should we even want to change someone’s DNA?
DNA is the blueprint that carries the instructions for the development and normal functioning of living organisms. Each cell in our body has the ability to assemble proteins based on the information contained in DNA. These proteins, in turn, carry out every imaginable function, from responding to stimuli to transporting cargo (i.e. molecules) across long distances and much more. Because DNA literally contains the information on how to assemble the building blocks of an organism, the ability to modify the code can have almost unlimited potential. This is called genetic engineering.
For instance, one of the most commonly discussed (and also controversial) uses of genetic engineering is in designing new plant species (commonly known as GMOs). This concept has been used to create plants which produce added vitamins (which may be missing from some people’s diets—see golden rice), plants which have longer shelf lives, better yields and much more.
Although modifying plants is one of the best-known applications, genetic engineering is also crucial in the manufacturing of certain types of medicine. Insulin for instance, used in the management of type 1 diabetes, is produced by genetically modified organisms. Indeed, bacteria (and recently even plants) have been modified to synthesise insulin in large quantities—so that it can be harvested from bioreactors. The same goes for many other products, such as human growth hormones and some vaccines.
And it doesn’t end there. Genetic engineering is also an invaluable tool for scientific research. Having the ability to modify DNA directly is one of the most important aspects of modern biology. Those white mice in cages? You guessed it, they’re genetically modified. In fact, there are entire libraries of mice that researches can choose from, which have been engineered to have any number of useful characteristics, such as organisms which can act as models of human diseases (i.e. mice that develop Parkinson’s disease). It can easily be argued that many advances in modern biology would not have been possible without the amazing toolbox that genetic engineering provides.
These colourful looking fish are the result of genetic modifications.
All of these things, however, already exist. We already live in a world where genetic engineering is a well established method used in countless industries (I mean, look at those genetically engineered glow-in-the dark fish you can buy, isn’t that straight out of a sci-fi movie?). Some worry about a world were genetic modification are pervasive, enabled by advances such as CRISPR. The truth is we already live in that world. CRISPR is simply the next step.
So why are we talking about this now, what does CRISPR change?
Although the CRISPR/Cas9 system could simply be dismissed as the latest tool in a long line of techniques for increasingly precise and efficient genetic manipulations, it is still a groundbreaking step because of its ease of use and low cost.
One of the biggest reasons everyone is getting excited is gene therapy, or the use of genetic engineering directly on humans for therapeutic reasons. With gene therapy, the stakes become much higher—potentially saving countless lives and relegating some of the worst pathologies to the history books. Being able to efficiently and precisely edit the genomes of living humans would unlock entire new approaches to curing illnesses. Just to give you an example, recent clinical trials have demonstrated that taking someone’s immune cells, genetically engineering them to recognise cancer cells and re-injecting them into the patient's body could slow down or even stop some forms of cancer. Although CRISPR is not strictly necessary to implement these new therapies, having a tool that is easier to use, faster to deploy and more precise could mean these treatments will arrive sooner and be accessible to a larger population.
And gene therapy doesn’t stop at re-engineering immune cells either. In fact, there are so many options that it would make little sense to list them all. For instance, some rare genetic diseases are caused by the lack of a specific gene. With CRISPR, one could simply add the missing sequence, essentially resolving the symptoms. This approach was used at the beginning of 2015 by a group of researcher, in a study that offers a striking illustration of the potential benefits of gene therapy. These scientists took monkeys infected with HIV and gave them a gene which encoded for a specific type of antibody. After the genetic treatment, the apes were cleared of HIV. These types of promising results are what turned genetic engineering (and CRISPR) into the buzzword it is today.
Enough with the scientific stuff, how this will shape the future?
The current applications of genetic engineering include some exciting prospects, such as improved crops, widely available therapies (insulin, etc) and gene therapy’s ability to rid us of countless pathologies. But what comes next?
With a sufficiently precise and reliable tool for genetic modifications in humans, like CRISPR appears to be, there is an almost infinite number of things one could imagine. Very quickly, we could move from eradicating medical conditions to enhancing humans. Once that door is open, almost anything is possible: faster growth, sharper senses, smarter minds—our genes determine everything we are, so changing them can affect anything we want.
At this point, an important distinction has to be made. There are two types of genetic modifications: those affecting somatic cells, which are cells cannot be transmitted further, and those affecting germline cells—which are passed on to future generations. Everything we have discussed in humans so far concerns somatic cells. Such modifications only affect the person receiving them, which means the risk is much more contained. In fact, many countries currently expressly forbid germline modifications. Still, the possibilities offered by modifying somatic cells are vast and promising—even if we do not veer into the dangerous territory of hereditable genetic modification, we will make great strides towards improving the human conditions and ushering in a better future for many.
But germline modification would offer even more potential. With designer babies, for instance, desirable traits could be inserted directly in embryos prior to implantation. This is something many worry about, because the consequences of introducing engineered genes in our collective human DNA are hard to predict, and therefore potentially dangerous. As is often the case, the high risk is also coupled with high reward. Modifying embryos, and therefore the human gene pool, could lead to the complete eradication of any trait which may be considered undesirable. Despite the undeniable potential, many scientists believe we should not attempt this until we develop a more precise understanding of the multifaceted interactions between modified genes and the organism.
Designer babies: could we soon be designing babies, choosing their characteristic from a catalog?
In an attempt to drive a sensible debate, some futurists have argued that such tools may end up only benefiting a small elite of wealthy individuals, leading to an ever increasing gap between the wealthy and genetically improved, and the rest. Clearly this is hard to predict, but if there is one undeniable fact, it is that gene therapy will lead to profound changes in our society. If we are not careful, it might easily take us down a dark and difficult path.
That’s both scary and amazing…but how much of this is just hype?
There has recently been widespread hype around CRISPR and genetic engineering in general. Usually, this kind of intense interest from the general public is not a very welcome thing for a scientific field. The unfortunate result of so much scrutiny is often loud alarmism and hasty conclusions—a misinformed exercise in wild speculation. Of course scientists should strive to disseminate their work and awaken the public’s interest, but when the subject is as polarising as it is with CRISPR, there is bound to be a lot of nonsense thrown around. This is reflected in a generalised exaggeration of both the benefits and the risks. As we have seen, CRISPR, and genetic engineering as a whole, will have a staggering impact on the future of almost all human activities, from the medical to the industrial. However, it is good to part with a slightly more realistic take—bringing us back from the clouds and onto solid ground. In a recent article, Jocelyn Kaiser of Science, one of the world’s leading scientific publications, argued that there are many substantial hurdles and potential setbacks that still need to be addressed before CRISPR can deliver on all the promises scientists have made. Although some may describe it as the holy grail of genetic engineering, others are more wary of hasty conclusions, especially when patients’ hopes are involved. As often in science, for now the consensus should be: cautious optimism.
With the recent controversy surrounding Tesla's autopilot crash, autonomous cars have been at the centre of attention. Of course, in that particular example, most of the controversy stems from a misunderstanding of what autonomous cars are, and what Tesla’s system is. Indeed, the Californian automaker’s approach is to provide the driver with support (think of it like a fancier version of cruise control). An autonomous car on the other hand, is one designed to get its passengers from point A to point B without any intervention from them (except for choosing the destination of course, otherwise you would get the premise for a Steven King novel, not a driverless car).
Truly autonomous transportation is already here. Some startups (BestMile for instance) have placed driverless vehicles on public roads. These are still in the early stages, and it may yet be a couple of years before these futuristic machine become a mundane sight. In all likelihood, we will start seeing public transportation transition to autonomous systems first (Mercedes just tested their driverless bus), followed by the release of fully autonomous private vehicles. The reason for this release order, is that public transportation is easier to solve from an engineering standpoint (fixed itineraries, repeatable scenarios, urban only, etc). In fact, one could even imagine "cheating" and using additional sensors placed along common itineraries to help guide buses around cities.
There is very little doubt that we will soon see the world transition to autonomous vehicles (and hopefully electric too, while we’re at it). These are three ways in which driverless cars will radically transform our lives.
They will give us our cities back
Cities all over the world are designed around transportation (and rightly so, considering we need to, you know, get there somehow)—roads and rails intertwined ad infinitum, mixed with a healthy dose of parking lots. These urbanistic constraints are a consequence of our modes of transportation, which are far from optimised. In fact, the general consensus is that the average car is parked 95% of the time, or roughly 8 hours of use per week—widely inefficient, considering there are many more cars then we would theoretically need. This is why cities are full of parking lots. We are so used to the sight, that we may not even pay attention to it, but our cities are literally filled with cars.
Can you count the parked cars in this picture? In the future doing so might be a lot easier…
This is set to change with the advent of autonomous cars. Fleets of driverless vehicles will carry passengers to and from their destinations, and will not need to wait idly in a parking lot until the same passenger needs a lift again. Instead, these cars will pick up anyone who might need it, at the tap of a finger (much like Uber, click here for Tesla’s plan to do exactly this).
Arguably, these autonomous cars will still need to park somewhere (to charge, and to avoid wasting energy when there is little need for them, such as in the middle of the night). However, these charging centres needn’t be scattered around city centres and residential neighbourhood, but could be placed in less conspicuous locations.
The impact this will have on the way our cities are organised will be profound. A lot of space will be repurposed and given back to the people. The cities of the future are likely to be a lot more human friendly and human-centric. Not only will parking spaces be dramatically reduced, but streets could also theoretically become narrower. This is because a fleet of autonomous cars will take up much less space than an equivalent number of human-driven cars. Since "smart" cars can communicate with each other and are not limited by slow human reflexes, they can move in highly optimised streams, with reduced distances between cars and matched speeds.
Rejoice: switching to autonomous cars will make our cities more beautiful and enjoyable to live in. Gone will be the endless parking lots.
They will give us a lot more time
Driving to work is something most people do not enjoy and understandably so, considering it’s essentially a big waste of time. While driving a car, you can’t do anything else (except perhaps listen to an audio book). It requires focus, and if we are being perfectly honest, is utterly boring. Yet many people spend huge amounts of time doing just that. The average American for instance, spends around 2 hours per week commuting, or roughly 100 hours per year (this includes all types of commutes, not just cars).
Consequently, one can compute that Americans collectively spend 3.4 million years commuting, every year. That’s a lot of “wasted” time, a lot of untapped potential. Of course, computing it this way is purposefully misleading, because multiplying a small amount of time with the population of a large country predictably gives a big number (like the fact that Americans collectively spend 200 thousand years brushing their teeth every year). Still, the point is that people spend a lot of time in their cars, unable to do any work (or to relax) because they have to focus on the task at hand.
Clearly, autonomous cars will radically change this, since any time spent in a car will essentially be free time (much more so than in a crowded metro or train). The interior of autonomous cars will look very different from the cars we are used to today. They will be designed like small living rooms, where the occupants can enjoy their travels, rather than a cockpit built around the controls. Some will use this time to catch up on some much needed sleep, others for leisure, and some for getting a head start to a long day’s work—the net result being a workforce which is more productive, more rested and generally happier.
Ironically, this will most likely lead to commutes getting longer on average, as they become less and less taxing.
They will save our lives
Cars are dangerous. A staggering 1.3 million people are killed worldwide in car crashes every year, and an additional 20-50 million are injured. Those numbers will seem absurd to future generations, for whom autonomous cars will be an extremely safe mode of transportation.
Most road accidents are caused by human error—switching to driverless cars will make them orders of magnitude safer. Regardless of the ethical and practical hurdles that we will need to overcome in the coming decade (questions like: who is responsible when a software bug causes a tragic bus crash?) the end result will be a dramatic reduction in mortality on the roads.
The good thing about the safety benefits, is that we won’t need to wait for fully autonomous vehicles to start reaping the rewards of smarter technology. Already today, Tesla’s cars appear to be a few times safer than regular cars when autopilot is enabled (although there is arguably not enough data yet to really draw any conclusions). With every software update and every new car model, they will only become safer. This trend will be similar to what happened with commercial aircrafts (where amongst other things, the introduction of autopilot made flights safer). To put things into perspective, if the rate of airplane accidents was still as high today as it was in 1973, there would be a fatal crash every two days (because the number of flights is much higher today, see here).
To conclude, driverless cars will have a profound effect on most aspects of our society. Not only will they save tens of thousands of lives, but they will also make our cities more liveable and give us back some much needed time. I don’t know about you—but me? I can’t wait!
Did you think this article was overly optimistic? Do you see any negative trends that could accompany the release of driverless cars or some other positive ones that weren’t mentioned? Share your thoughts in the comments below!
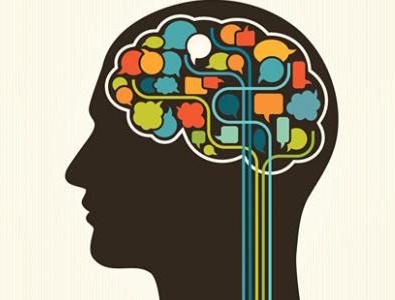
The existence of free will is a classical question in philosophy, and one which has been around for a while (unless you consider Greek antiquity the recent past). Unlike some of the more far-fetched thought experiments philosophers like to indulge themselves in, free will is something everyone can relate to, and about which everyone seems to have a strong opinion. This arguably makes it hard to approach the question rationally, considering everyone starts with a deeply rooted prior position.
Before we dive into the thick of the subject, we need to define a few things. Let’s start with epiphenomenalism, which is the belief that conscious experiences are a by-product of our brains’ activity. Adherents of this view state that the brain's activity is the sole cause of any observable behaviour, and that conscious emotions cannot influence the output of the system. To borrow Thomas Huxley’s words: “consciousness is completely without power…as the steam-whistle which accompanies the work of a locomotive engine is without influence upon it’s machinery.” In this framework, we might think we cry because we are sad, but in reality, there is a common cause to both events. Something made us cry, and that same thing gave rise to the conscious experience of sadness, thus creating the impression that we cry because we are sad, when in reality we cry for the same reason we feel sadness, whatever that reason may be. Although epiphenomenalism is the fancy name for it, this is identical to saying humans are automatons, responding to external stimuli in deterministic ways.
Another concept which needs defining is qualia. Simply put, a quale is "the way it feels” to experience something. For instance, the subjective experience of pain is a quale. If one were to describe the feeling of pain to another person, no matter how precise the description, the listener could never experience the quale associated with it, and would certainly learn something new about pain once he experienced it for the first time.
Okay, now that the defining is out of the way, let’s get to it.
The following reasoning, which is anchored in our current understanding of the brain and the nervous system (limited as it may be), is, in my opinion, the strongest argument for epiphenomenalism yet. It goes like this: the only way humans can interact with the outside world is through the motor system—the nerves and muscles controlling our movements, speech and everything else. If we follow the chain of events leading to any motor action, we will find that the muscle contraction responsible for the movement was the result of the firing of a motor-neuron (a type of nerve cell). If we trace that neuron backwards, we will find that it originates somewhere (perhaps in the spinal cord, or in the brain). At this point, we may ask ourselves why that neuron fired. We know from neurophysiology that a neuron fires whenever all the other neurons which are connected to it manage to affect its voltage sufficiently (depolarise it and trigger an action potential). So in order for that specific motor neuron to be active, some of the ones connected directly to it had to be active before. In turn, those neurons themselves could only be active if their own partners were—you can start to see where this is going. If we continue following the chain of events, we can explain all of the activity a person produces as a logical (and inevitable) consequence of the past state of all the cells in his or her brain, spinal cord and nerves.
A neuron’s dendritic (input) tree. This “tree” allows the neuron to create contacts (synapses) with a large number of other cells.
In fact, there is a growing body of empirically evidence supporting this view. The readiness potential (first reported in 1964) is a stereotypical activity which can be measured in specific brain areas (such as the motor cortex). What makes this particular type of activity interesting, is that it has repeatedly been shown to precede the conscious decision to move. Some scientists have even designed an experiment in which a computer predicts whether or not a subject will press a button, before they do so (and more importantly, even before they consciously decide to do so). Of course such studies still have some weaknesses (for instance, how does one precisely determine the point in time where a conscious decision is taken?) Still, these types of results are certainly enough to give pause.
This seems to lead to the inevitable conclusion that free will cannot exist. Any quale, any conscious experience, must either be a side product of the brain’s activity (like the whistle of the locomotive), or perhaps, an intrinsic property associated with the state of the network of cells in the brain (this view is called materialism, or physicalism). However, these subjective experiences cannot shape the outcome in any way.
In order for any characteristic to survive the process of natural selection, it should confer an advantage in terms of survival.
Of course, this raises the question: why do we have consciousness at all, if it is useless? In other words, we might as well be philosophical zombies (a philosophical zombie is a being which acts exactly like we would expect a human to, but does not experience qualia, or any type of conscious experience). This is of course a very difficult question to answer. Indeed, it would seem odd that consciousness exists at all, if it provides no evolutionary advantage. One possible answer to this conundrum, which has recently become popular again (owing in part to the work of Giulio Tononi and Christoph Koch), is panpsychism, or the idea that consciousness may actually be an intrinsic property of the universe (like mass). In this view, every object has some degree of “consciousness”. Of course, a rock may have little (or perhaps none), while a structured group of cells (like a brain) may have a lot. The reason this theory is interesting, is that it helps solve the evolutionary argument: if it is impossible to have a system which processes information, without having an associated subjective experience, then it would follow that as evolution selects for increasingly complex organisms, these organisms must automatically have a growing amount of consciousness and subjective experiences. This is exactly what Giulio Tononi tries to formalise in his integrated information theory of consciousness (I encourage you to read more about this fascinating science here).
In Giulio Tononi’s theory, how tightly connected a set of nodes are determines how high their consciousness (or phi) is.
In practical terms however, the brain is a chaotic system—meaning that although it is most likely purely deterministic in nature, predicting the outcome is not feasible on any relevant time scale. Like the weather, it would be impossible to predict a person’s actions based on the current state of his brain, for more than the immediate future. This leads to the following comforting conclusion: we do not have free will, yet nobody can predict what we will do next (outside of some specific “press the button” types of situations).
One of the most popular arguments against epiphenomenalism (or any theory which argues for the non-existence of free will) is the “obvious absurdity” argument—it is plain for everyone to see that we have free will, since we constantly take decisions and execute actions according to our own desires. This argument, although very emotionally convincing (like when the old professor suddenly lifts his arm in the air and exclaims: “no free will my ass!”), entirely side-steps the problem. If epiphenomenalism is true, and we are under the illusion that our conscious experiences precede and cause our physical actions, then we would by definition be under the illusion of having free will. Any individual’s conviction of possessing free will is therefore not evidence for, nor against, either position. To paraphrase B. de Spinoza's pithy remarks, we are under the illusion of freedom simply because we are conscious of our actions, and unconscious of the causes whereby those actions are determined.
There may yet be one way to escape the inevitable conclusion that free will does not exist: quantum physics. If we look at the behaviour of very small systems (atoms, molecules, etc.), the classical laws of physics we are accustomed to at our macroscopic scale start breaking down. In the world of the tiny, matter behaves in a fundamentally different way. It behaves according to statistical laws, which means some questions cannot be answered precisely, but only in terms of probabilities. For instance, it is impossible to predict when a radioactive atom will decay. This means that at such small scales, determinism has some wiggle room. This wiggle room is where the theories known collectively as quantum consciousness come into play. Indeed, some scientists argue that the processes underlying the brain’s function are subject to the laws of quantum mechanics (because neurons operate at a scale where such phenomena are relevant). That the brain is subject to quantum effects does not, per se, tell us anything about free will. However, some have argued that free will may be introduced into the system by playing with the probabilistic nature of quantum mechanics. In this far-fetched view, the outcome is not altered on average, but free will intervenes by changing the outcome at the level of single quantum events. To give a simple analogy, this is like saying that free will may change the number we get when throwing a dice, but on average, each number must still appear in equal proportions. So if we force a specific number to appear now, we must compensate for it later. This very limited wiggle room may still be enough to give us a small nudge in the direction of free will.
The great physicists of the past century may ultimately hold the key to free will.
So far, none of the quantum brain theories have gained widespread adoption (mainly because there are no convincing ones yet). Still, quantum mechanics may be regarded as the last hope for those trying to prove the existence of free will.
I hope this article has awakened your curiosity about the subject of free will. If it has, I encourage you to read up on it to get a more balanced view of the question. If you find something interesting, do come back and share it in the comments!
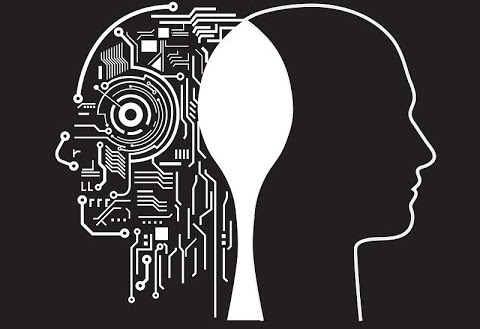
Bringing up the singularity—the point of no return after which our feeble human minds are unable to even fathom what the future may bring—is a sure-fire way to ensure the fun engineering party you’re attending (what? a fun engineering party? invite us next time!) ends in a passionate feud. This seemingly all pervasive topic is a favourite amongst the technogeek elite—the sic-fi writers, futurists, engineers and electric-car-building-billionaires of this world. In more practical terms, the singularity is often defined as the point where artificial intelligence (AI) algorithms become more capable than their human creators.
There are two prevailing opinions regarding what would happen after the singularity (hence the passionate feud). The first view, which is very optimistic, goes a little like this: once sentient machines become smarter than us, we must trust that they will generously continue helping us to achieve our goals (cure illnesses, improve our quality of life, etc). Of course, the defenders of this approach will argue that we can make sure machines will always have our best interest in mind by including some basic rules deep within their design (such as Asimov’s three rules of robotics). The second view is—how shall we put this lightly—slightly more pessimistic. According to this version of events, sentient machines will realise we are not helpful to them (or worse, harmful to them) and destroy us, enslave us or otherwise harm us. This is the Terminator style apocalypse, and it sells well (to be fair, the movie where the robots and humans live happily ever after would never make a big splash at the box office). Hopefully, by the time you reach the end of this article, you will disagree with both views.
But wait, you may ask, before I start preparing my house for the machine apocalypse (though if you are, remember to pack electromagnets), are we even sure this will happen? As often when the matter at hand involves predicting the future, there are conflicting reports. Ray Kurzweil, author of The singularity Is Near, believes that, well, the singularity is near. More seriously, he narrows it down to the year 2045, give or take. Others, like the scientist and author Steven Pinker, dismiss the idea altogether. Some of the criticism about the concept of a technological singularity is very reasonable and embodies the kind of scientific skepticism which should be revered. For instance, some argue that technological progress will not indefinitely follow an exponential growth, but may on the contrary slow down as each further improvement becomes harder because of increasing levels of complexity and knowledge (this is known as the complexity brake).
Regardless of how we look at the problem, I think it is only fair to assume that mass extinction aside, we will inevitably develop artificial intelligence on par, or superior, to our own. It may take longer than expected, much longer, but that does not prevent it from happening. To argue that it is impossible to develop an algorithm smarter than ourselves is likely a common, although misguided, type of anthropocentric wishful thinking. There is no escaping it—the singularity, as in machines which are smarter than humans, will happen sooner or later.
Should we prepare? Can we prepare? That would probably depend on which side of the argument you stand, the optimistic side, or the apocalyptic one. I believe the singularity won’t matter, because by then, we will be so intimately merged with machines, that it won’t be us vs them. Instead, the singularity will directly benefit us. With our current technology, it makes a lot of sense to distinguish between humans, and the tools they use—the machines. But in the future, as we develop increasingly complex and efficient brain to machine interfaces, the distinction will start to blur. We will have a tight symbiotic relationship with our technology, allowing us to take full advantage of it. By that time, asking about the singularity won’t make much sense anymore. The evolution of the human mind and its capabilities will be a progressive process, and the day we become incrementally smarter, thanks to our merger with computers, will not be a significant day at all. I once read this “mind-blowing fact” somewhere: there is a specific day in your life, when your mother carried you in her arms for what was to be the last time, yet that day bore no particular importance, and nobody noticed it. The singularity will be similar. We may see it thinking back, decades later, maybe pin down the year it happened, but it will carry no special significance as it unfolds.
Of course, there is still the possibility that we will develop super-intelligent AI before we have the technology to seamlessly interface ourselves with computers. If that were to happen, then we may find ourselves faced with the common dilemma we discussed earlier. Both areas (AI and neuroprosthetics) are almost unimaginably complex. Both have captured the imagination of thousands of scientists and engineers around the world. Both have spawned enormous and very active fields of research. And in many instances, there is a fair amount of overlap (AI is used on a daily basis in neuroprosthetics, and the study of the brain is often key to designing novel AIs). It thus seems reasonable to assume that they will continue to develop in parallel, and that extreme advances in one will not happen without similar advances in the other.
Although the singularity is inevitable, it won’t be something to worry about. In fact, we won’t even notice it.
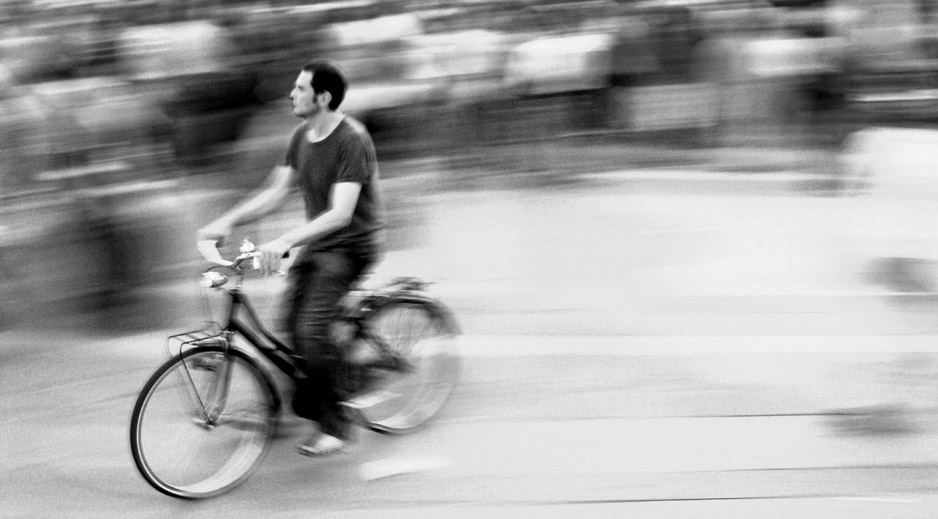
In 1981, Steve Jobs famously compared computers to “bicycles for the mind”—although in all likelihood he meant only Apple computers. The metaphor was born when he chanced upon an interesting chart, showing the efficiency of locomotion for various animals (the energy consumed to cover a certain distance). Although Steve later claimed humans were mediocre in terms of efficiency, the truth is that humans are amongst the best walkers in the animal kingdom (a direct consequence of bipedal locomotion, allowing humans to save copious amounts of energy from step to step—this is called inverted pendulum walking). Still, humans are amongst the worst sprinters, and fairly lousy long distance runners (charts). Regardless of where we stand in the charts, human ingenuity has allowed us to transcend our physical limitations, and soar high above all animals with one simple invention: the bicycle.
This is a very striking illustration of the power of human minds—allowing us to invent solutions to overcome most of our physical shortcomings. We thrive in environments we are not suited for, and repeatedly outsmart animals who would otherwise overpower us. The reason Steve’s metaphor is so compelling, is the suggestion that computers might increase the efficiency of our thinking like bicycles improved the efficiency of our walking. Being able to enhance the way our minds function would be a key turning point, leading to a staggering snowball effect, where smarter minds are able to enhance themselves further, in a dizzying spiral of increasing intelligence.
Sadly, I believe we can all agree: the personal computer revolution Steve helped pioneer did not produce the bicycle for the mind. Sure, computers may be the most important tool we have ever built—together with the internet—allowing us to connect, share and create knowledge in new ways, much like the invention of print did centuries ago. In science, computing has allowed the creation of entire fields of research and unprecedented mathematical prowess. Still, that is not enough to qualify as a bicycle for the mind. Despite making us more efficient during certain tasks, such as searching for information, or tackling specific problems, computers do not enhance our minds in a pervasive, generalised way. Perhaps computers are more akin to the invention of the wheel—a necessary stepping stone towards the creation of the bicycle, and a useful tool in itself—but carrying a wheel in your backpack does not make you walk any more efficiently (not that I have tried, mind you).
In my opinion, the main reason computers fall short of Steve's vision is our extremely limited ability to interact with them. Our minds operate at the blistering speed of thoughts. Yet, when using a computer, we cannot send instructions at our usual speed—we have to rely on a much slower approach, dragging a pointer around an interface and clicking on things, or other similarly clumsy artifices. As many frustrated users will attest, operating computers this way can be painfully slow at times, and many tasks which our minds can envision and plan out in a matter of milliseconds, may take dozens of seconds to physically execute on a machine. Technically, this means the communication between our minds and computers is low bandwidth (particularly in the brain to computer direction), preventing us from truly developing a symbiosis with them.
In order for a wheel (or two) to become a bicycle, someone had to think of a way of interfacing human bodies with this useful invention. In that case, the answer was to place a person directly above a set of wheels, where the pedals act as an optimised physical interface, drawing from the natural motion of the legs to thrust the bicycle forward. The same holds true for computers. In order for our minds to truly take advantage of them, we need to invent an interface between the mind and the machine allowing for seamless exchange of information—in other words, the pedals.
For our minds to take full advantage of computers, they need to be able to interact at their natural speed, and in their natural language—thoughts. Imagine being able to control your phone, or your laptop, by simply thinking about it. For instance, opening a photo, changing the brightness and renaming it would be a breeze—no fiddling with menus and settings. Similarly, performing an internet search would no longer require opening a browser, then a new page, and typing the query before hitting enter. Instead, a simple thought would immediately bring up the corresponding answer on screen. This way, computers would become an extension of the mind, rather than a mere tool.
We could even take this one step further—albeit by unquestionably entering the realm of science fiction: what if, instead of bringing up the answer on a screen, it was sent back to our brain directly in the form of a thought. Imagine wondering about a question, and instead of seeing symbols appear on a screen, simply suddenly becoming aware of the answer. Now that, is what I would call a bicycle for the mind.
Knowing what the bicycle is, the more pressing question becomes: when will I have one? The simple answer: sooner then you might imagine.
The missing puzzle piece to achieve this vision is an interface providing a seamless link between the mind and the machine. Such technologies already exist—we have the ability to record the brain’s activity with relative precision, and extract a bunch of information from it—but they still present many issues which need to be addressed. To illustrate how far we have already come, one simply needs to look at the recent clinical trials where such interfaces allowed paralysed patients to control robotic arms, type on a keyboard or play games directly with their minds (video). Still, solving these complex issues may well take us another decade or two, but the cold and inexorable progress of science ensures that we will get there eventually. Once we have the interface, it will only be a matter of putting the pedals and the wheels together.
When Steve jobs envisioned the bicycle for the mind, he wasn’t wrong—he was simply half a century too early.
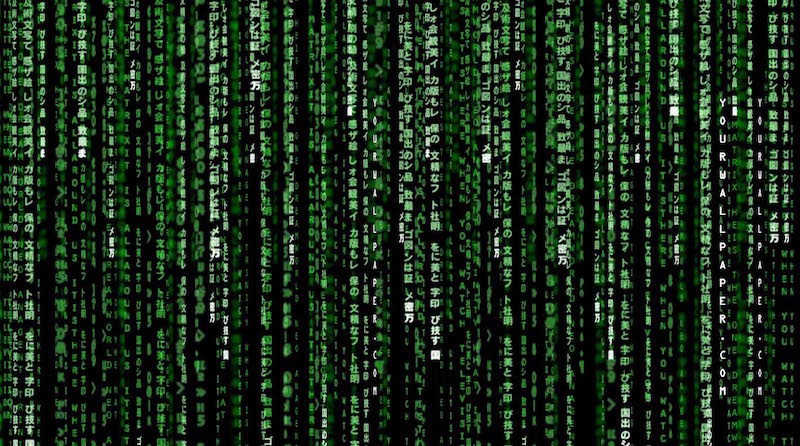
In the 1999 classic the matrix, the Wachowski Brothers depicted an alternative reality in which all humans are connected to a central machine (the matrix) feeding them a form of hyper-realistic virtual reality, which only exists in their collective minds. By keeping them in this state, sentient machines are able to harvest the energy produced by each person to feed their own power needs.
The thought is disturbing. How would someone know whether what he sees is an illusion, instead of reality? The answer: it’s impossible. As Descartes famously said, the only thing one can be sure about is that one is indeed a thinking entity, everything else may very well be a trick. And this fact alone, in my opinion, makes the plot so compelling. Because as the movie reveals its secret, we are brought to reflect on our own condition, and we cannot help but conclude that we too, may be part of a matrix.
In fact, I believe most of us are already living in a version of the matrix today. Let me explain why.
The world has changed radically in the last decades. The invention and subsequent mass adoption of the internet has transformed almost every aspect of our lives in a profound way. It is the most significant revolution in the way humanity shares, seeks and generates knowledge since Gutenberg invented the printing press over half a millennia ago. The internet has become our main source of data. For most millennials it might seem odd to use other means to find information on a day to day basis. We use it to read the news. We use it to search for snippets of knowledge. In fact, almost all of the new information we acquire throughout the day comes from the internet (from our social networks, wikipedia, search engines and other places). Ironically, when we interact with each other in person, we usually share information we obtained online (did you see what Chris posted on Facebook? Did you hear the news about Italy?).
This is not, per se, a bad thing. Though this is a matter of opinion. It is simply the way of our modern world. Some may cling to the past, regretting better days where one could be blissfully ignorant of facts, without someone grabbing his hand-held computer and finding the relevant piece of information more quickly than it takes to change the subject. But there is no going back, and the internet will inevitably play an increasingly central role in our lives.
So far so good you might say, why all the fuss about the matrix? Well, your patience is about to pay off. The problem here is subtle. The problem is that there is no such thing as the internet (as in a single unified entity). We interact with the internet in very stereotyped manners: we either load up a search engine (most likely Google) and type in our query, or we navigate directly to our website of interest (most likely Facebook) and browse the content available there. However, in an effort to improve our experience in the online world, these companies are resorting to a very smart trick: they are tailoring the results and the content to better match our tastes and expectations. This is not new, and this is not secret. It is however, something many people are unaware of. The underlying motives are good: users are provided with a more enjoyable experience, and the search engines (or social networks and others) receive more traffic from happy users. It’s what one may call a win-win. Except it isn't.
How could this type of customisation be harmful? Let me give you a simple example. When I search for the keyword python, the first five pages do not mention anything about snakes or english comedians. Because Google has learnt that I am interested in programming, it helpfully brings up lots of pages about coding, hiding away what I am probably not looking for (Monthy Python or python snakes). On the other hand, when I do the same search anonymously, a menacing snake appears on the first page.
This becomes slightly disturbing when we consider the implications. If I had never heard of the British comedians Monthy Python, or about the snake, I might never chance upon them. I would remain unaware of their existence. This creates a sort of vicious cycle, where we are fed what we look at most, and automatically look at more of what we are fed. It becomes harder to chance upon new things, new ideas. By feeding us the content we are looking for, these services are also hiding away the content we aren’t looking for.
This was a rather harmless example, but one can easily see how it might be more pernicious when talking about politics, history or conspiracy theories.
Of course, humans have an innate tendency to do this on their own. People will often seek out others with similar opinions, or interacts with groups who share their ideals. Reinforcing this tendency by customising our access to the internet capitalises on this natural drive.
At the end of the day, these services contribute to the creation of what we may call a personality inertia, where people with a certain personality are encouraged to move forward in that direction, and where it becomes increasingly arduous for someone to diversify and expand.
Not all is dark however. Google for instance, is aware of this and tries to prevent it to some extent (by mixing in results they think you will like with some “untainted” results). But they might be the most benevolent in the bunch (do no evil, right?), what about news aggregators? What about Facebook, or Youtube? Can we truly believe all of these services are sacrificing some traffic for the sake of diversity?
Our digital world is a prison of sorts. Like the humans in the matrix, we are shown an illusion of the real world, a version of the real world designed to keep us happy, to reinforce us in our beliefs and sentiments.
This brings us to the following spooky conclusion: what you see when you look at your monitor, is not this beautiful, interconnected mesh of minds so many call the internet. Rather, what you see is a version of the internet tailored specifically for you, which in many ways is simply a reflection of your own personality.
In an interesting twist of events, if we dig deeper, we discovers that the way this customised internet is built and tailored for each one of us is through the use of machine learning algorithms. It turns out that what is keeping us in this virtual world, drawing one final and uncanny parallel to the movie, is precisely the same thing that was keeping the people from the movie trapped in the matrix: artificial intelligence. Luckily not the energy harvesting kind – for now.